Automated Machine Learning (AutoML) Market by Offering (Solutions & Services), Application (Data Processing, Model Selection, Hyperparameter Optimization & Tuning, Feature Engineering, Model Ensembling), Vertical and Region - Global Forecast to 2028
The global Automated Machine Learning Market size accounted for USD 1.0 Billion in 2023 and is estimated to achieve a market size of USD 6.4 Billion by 2028 growing at a CAGR of 44.6% from 2023 to 2028. Transfer learning is an important aspect of AutoML that leverages pre-trained models to improve the performance of new models. By transferring the knowledge learned from one task to another, businesses create more accurate models with less training data and reduce the time and cost required to build high-performing models.
To know about the assumptions considered for the study, Request for Free Sample Report
To know about the assumptions considered for the study, download the pdf brochure
Automated Machine Learning Market Growth Dynamics
Driver: Rising need to transform businesses with Intelligent automation using AutoML
As businesses increasingly rely on data to drive decision-making and improve operational efficiency, a growing demand for intelligent business processes has been growing. These processes leverage machine learning algorithms to automate decision-making and optimize business operations, leading to improved performance and increased profitability. By leveraging AutoML, businesses can streamline operations, reduce costs, and improve performance, ultimately resulting in a competitive advantage. According to a report by industry experts, it is found that AI-driven automation can improve productivity by up to 40%. Automated Machine Learning Market can help businesses achieve these kinds of results by automating the development and deployment of machine learning models. By using AutoML, businesses can quickly and efficiently develop predictive models that can be integrated into existing business processes. These models can then be used to automate decision-making, optimize processes, and improve performance. Additionally, AutoML can help businesses identify new opportunities for optimization and improvement that were previously difficult to detect. By analyzing large volumes of data, AutoML can identify patterns and trends, enabling businesses to make data-driven decisions to improve performance and drive growth.
Restraint: Machine learning tools are being slowly adopted
The slow adoption of automated machine learning (AutoML) tools is a significant restraint hindering the growth of the AutoML market. Despite the numerous benefits AutoML offers, including increased efficiency, accuracy, and scalability, many organizations hesitate to adopt this technology. One of the primary reasons for this slow adoption is a lack of awareness about Automated Machine Learning (AutoML) Market and its capabilities. A survey by O’Reilly found that only 20% of respondents reported using automated machine learning tools, while 48% had never heard of the technology. This lack of awareness is a significant barrier to its adoption, as many business leaders and decision-makers may not be familiar with the benefits of AutoML and the impact it can have on their business.
Another factor contributing to this slow adoption is a shortage of skilled data scientists and machine learning experts. According to a report by an industry expert, there will be a shortage of up to 250,000 data scientists by 2024. This shortage of skilled experts can make it challenging for organizations to develop and deploy machine learning models, leading to slower adoption rates. Concerns about the transparency and interpretability of machine learning models are also holding back adoption. This lack of transparency can be a barrier to adoption in industries such as healthcare and finance, where decisions based on machine learning models can have significant consequences.
Opportunity: Seizing opportunities for faster decision-making and cost savings
The increased accessibility of machine learning presents a significant opportunity for the AutoML market. Historically, machine learning has been highly specialized, requiring extensive statistics, programming, and data analysis expertise. However, with the development of AutoML tools, businesses no longer need to have a team of data scientists and machine learning experts to build and deploy AI solutions. Instead, AutoML tools enable businesses to democratize the use of machine learning, making it more accessible to a broader range of users and use cases. By using AutoML tools, businesses can quickly build and deploy predictive models that can analyze large amounts of data and identify patterns, anomalies, and insights that may not be apparent to the human eye. For instance, AutoML models can help businesses to predict customer behavior, optimize pricing strategies, and identify opportunities for process improvement.
Moreover, the increased accessibility of machine learning can also lead to significant cost savings for businesses. By using AutoML tools, businesses can reduce the costs associated with hiring specialized talent and investing in expensive infrastructure. Additionally, the faster development and deployment of AI solutions can result in cost savings by increasing operational efficiency and improving decision-making. Furthermore, the increased accessibility of machine learning can also lead to new business innovations and opportunities. As more businesses adopt AutoML tools, there is likely to be a proliferation of new use cases and applications, leading to increased innovation and growth in the market. Additionally, the democratization of machine learning can enable businesses to tap into new markets and expand their offerings, leading to increased revenue and market share.
Challenge: Increasing shortage of skilled talent
One of the biggest challenges facing the AutoML market is the shortage of skilled talent. AutoML platforms require individuals with a strong machine learning, data science, and programming background. However, the demand for these skills has far outpaced the supply, leading to a significant talent shortage in the industry. As a result, organizations struggle to find the right talent to build, deploy, and maintain AutoML models. According to a report by LinkedIn, data scientists and machine learning engineers are among the top emerging jobs in the technology sector. However, the shortage of skilled talent has led to fierce competition among organizations for a limited pool of candidates. The LinkedIn report found that data scientist positions take an average of 42 days to fill, highlighting the challenges of finding qualified candidates.
The rapid pace of technological advancements further compounds the shortage of skilled talent. As new algorithms and techniques are developed, it is essential for individuals working with AutoML platforms to continually upskill and stay up to date with the latest advancements in the field. This requires ongoing training and professional development, which can be costly and time-consuming. Furthermore, the shortage of skilled talent is not limited to data scientists and machine learning engineers. AutoML platforms also require individuals with expertise in areas such as data management, data visualization, and cloud computing. The talent shortage in these areas can also impact the successful implementation and adoption of AutoML solutions.
By application, data processing to account for the largest market size during the forecast period
AutoML can be applied to automate various aspects of data processing, including data cleaning, data normalization, and data transformation. Automated Machine Learning (AutoML) Market can automate the process of detecting and correcting errors in the data. This includes identifying missing values, correcting data formatting errors, and removing outliers that could affect the accuracy of machine learning models. AutoML involves techniques such as standardization and normalization, which can be automatically applied to the data. In transformation, data can be transformed to a more appropriate format, reducing the risk of errors and inconsistencies with the help of AutoML. AutoML can also integrate data from multiple sources, which is often a time-consuming and complex task. This includes techniques such as data merging and joining. By automating the tasks, AutoML can reduce the time and effort required for manual data processing, improving the quality and accuracy of the resulting data.
By deployment, cloud to account for the largest market size during the forecast period
The adoption of cloud computing has increased over the past few years, with internet connections becoming more reliable and remote work becoming the norm. Cloud-based AutoML solutions offer greater flexibility and scalability than on-premises solutions, as they can be easily scaled up or down as needed to accommodate changes in workload or data volume. Cloud-based solutions also typically offer a pay-as-you-go pricing model, which can be more cost-effective for organizations with variable workloads. Installing and supporting traditional software can be very challenging for a decentralized team. However, with cloud-based software, anyone can access the solution remotely. For SMEs, cloud is particularly useful as it provides full functionality at a reasonable rate and requires no upfront investment. Due to its commercial benefits and the world of possibilities, cloud technology has become a popular model for modern automation businesses.
By offering, solutions to account for the largest market size during the forecast period
AutoML solutions are designed to automate the tasks involved in developing and deploying machine learning models. This makes it easier for organizations to leverage the power of machine learning without requiring significant expertise in data science or machine learning. AutoML solutions are becoming an increasingly important tool for organizations looking to leverage the power of machine learning to gain insights from their data and make better decisions. By automating many tedious and time-consuming tasks involved in model development and deployment, AutoML platforms can help organizations accelerate their digital transformation and unlock new opportunities for growth and innovation. AutoML solutions are available in different types and deployment options. By type, AutoML solutions can be classified into AutoML platforms and AutoML software. By deployment, AutoML solutions can be deployed on-premises or on cloud.
North America to account for the largest market size during the forecast period
North America is expected to have the largest market share in Automated Machine Learning. North America has been a major contributor to the development and growth of the Automated Machine Learning market. The US is one of the most developed countries in the region. AutoML is a rapidly growing market in the US, with several key players offering solutions that range from fully automated platforms to ones that assist data scientists in building machine learning models. The market is being driven by the need for faster and more efficient ways to build and deploy machine learning models, as well as the increasing demand for artificial intelligence solutions in various industries. In recent years, there has been a significant increase in the adoption of AutoML solutions in the US, especially in industries such as healthcare, finance, and retail. Healthcare providers are using AutoML to analyze medical images and identify patterns in patient data, while financial institutions are using it to detect fraudulent transactions and assess credit risk. Retailers are using AutoML to personalize recommendations and improve customer engagement.
Key Market Players
The Automated Machine Learning Market vendors have implemented various types of organic and inorganic growth strategies, such as new product launches, product upgradations, partnerships and agreements, business expansions, and mergers and acquisitions to strengthen their offerings in the market. The major vendors in the global market for Automated Machine Learning IBM (US), Oracle (US), Microsoft (US), ServiceNow (US), Google (US), Baidu (China), AWS (US), Alteryx (US), Salesforce (US), Altair (US), Teradata (US), H2O.ai (US), DataRobot (US), BigML (US), Databricks (US), Dataiku (France), Alibaba Cloud (China), Appier (Taiwan), Squark (US), Aible (US), Datafold (US), Boost.ai (Norway), Tazi.ai (US), Akkio (US), Valohai (Finland), dotData (US), Qlik (US), Mathworks (US), HPE (US), and SparkCognition (US).
Want to explore hidden markets that can drive new revenue in Automated Machine Learning (AutoML) Market?
Scope of the Report
Want to explore hidden markets that can drive new revenue in Automated Machine Learning (AutoML) Market?
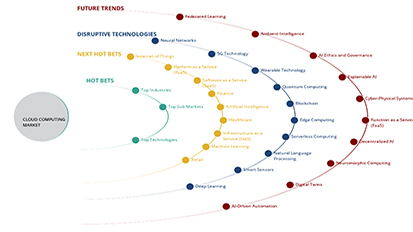
Report Metrics |
Details |
Market size value in 2023 |
USD 1.0 billion |
Revenue forecast in 2028 |
USD 6.4 billion |
Growth rate |
CAGR of 44.6% from 2023 to 2028 |
Market size available for years |
2017–2028 |
Base year considered |
2022 |
Forecast period |
2023–2028 |
Forecast units |
USD Million/Billion |
Segments covered |
Offering, Application, Vertical, and Region |
Geographies covered |
North America, Europe, Asia Pacific, the Middle East & Africa, and Latin America |
Companies covered |
IBM (US), Oracle (US), Microsoft (US), ServiceNow (US), Google (US), Baidu (China), AWS (US), Alteryx (US), Salesforce (US), Altair (US), Teradata (US), H2O.ai (US), DataRobot (US), BigML (US), Databricks (US), Dataiku (France), Alibaba Cloud (China), Appier (Taiwan), Squark (US), Aible (US), Datafold (US), Boost.ai (Norway), Tazi.ai (US), Akkio (US), Valohai (Finland), dotData (US), Qlik (US), Mathworks (US), HPE (US), and SparkCognition (US). |
This research report categorizes the Automated Machine Learning (AutoML) Market based on Offering, Cloud Type, Technology, Organization Size, Vertical, and Region.
By Offering:
-
Solutions
-
By Type
- Platform
- Software
-
By Deployment
- Cloud
- On-premises
-
By Type
-
Services
- Consulting Services
- Deployment & Integration
- Training, Support, and Maintenance
By Application:
- Data Processing
- Feature Engineering
- Model Selection
- Hyperparameter Optimization & Tuning
- Model Ensembling
- Other Applications
By Vertical:
- Banking, financial services, and insurance
- Retail & eCommerce
- Healthcare & life sciences
- IT & ITeS
- Telecommunications
- Government & defense
- Manufacturing
- Automotive, Transportations, and Logistics
- Media & Entertainment
- Other Verticals
By Region:
-
North America
- US
- Canada
-
Europe
- UK
- Germany
- France
- Italy
- Spain
- Nordic
- Rest of Europe
-
Asia Pacific
- China
- Japan
- India
- South Korea
- Australia & New Zealand
- ASEAN
- Rest of Asia Pacific
-
Middle East & Africa
- UAE
- Kingdom of Saudi Arabia
- Israel
- Turkey
- South Africa
- Rest of the Middle East & Africa
-
Latin America
- Brazil
- Mexico
- Argentina
- Rest of Latin America
Recent Developments:
- In February 2023, IBM integrated StepZen's technology into its portfolio, with the aims to provide its clients with an end-to-end solution for building, connecting, and managing APIs and data sources, enabling them to innovate faster and generate more value from their data.
- In February 2023, AWS launched new features for Amazon SageMaker Autopilot, a tool for automating the machine learning (ML) model creation process. The new features include the ability to select specific algorithms for the training and experiment stages, allowing data scientists more control over the ML model creation process.
- In October 2022, Oracle partnered with NVIDIA, which enabled Oracle to offer its customers access to Nvidia's GPUs for use in machine learning workloads, enhancing the performance and capabilities of Oracle's machine learning tools.
- In February 2022, AWS partnered with Maple Leaf Sports & Entertainment, which enables MLSE to assist its teams and lines of business. MLSE also employs AWS's extensive cloud capabilities, including AutoML, advanced analytics, computing, database, and storage services.
- In December 2020, Salesforce and Google Cloud announced a collaboration to integrate Google Cloud's automl tools with Salesforce's Customer 360 platform. The collaboration aimed to help businesses build a complete view of their customers and use AI to personalize their experiences.
Frequently Asked Questions (FAQ):
How big is the Automated Machine Learning Market?
What is growth rate of the Automated Machine Learning Market?
What are the key trends affecting the Automated Machine Learning Market?
- Data Processing
- Feature Engineering
- Model Selection
- Hyperparameter Optimization & Tuning
- Model Ensembling
Who are the key players in Automated Machine Learning Market?
Who will be the leading hub for Automated Machine Learning Market?
What are the opportunities in Automated Machine Learning Market?
- Banking, financial services, and insurance
- IT & ITeS
- Telecommunications
- Manufacturing
- Automotive, Transportations, and Logistics
- Media & Entertainment
To speak to our analyst for a discussion on the above findings, click Speak to Analyst
Exclusive indicates content/data unique to MarketsandMarkets and not available with any competitors.
- 5.1 INTRODUCTION
-
5.2 MARKET DYNAMICSDRIVERS- Growing demand for improved customer satisfaction and personalized product recommendations through AutoML- Increasing need for accurate fraud detection- Growing data volume and complexity- Rising need to transform businesses with intelligent automation using AutoMLRESTRAINTS- Slow adoption of machine learning tools- Lack of standardization and regulationsOPPORTUNITIES- Growing demand for AI-enabled solutions across industries- Seamless integration between technologies- Increased accessibility of machine learning solutionsCHALLENGES- Growing shortage of skilled workforce- Difficulty in interpreting and explaining AutoML models- Rising threat to data privacy
-
5.3 CASE STUDY ANALYSISREAL ESTATE- Case Study 1: Ascendas Singbridge Group improved real estate decision-making by leveraging DataRobot’s AutoML platform- Case Study 2: G5 employed H2O.AI’s driverless AI platform to address challenges in identifying productive leadsBFSI- Case Study 1: Robotica helped Avant automate key processes and streamline lending operations- Case Study 2: Domestic and General partnered with DataRobot to improve customer service capabilities- Case Study 3: H2O.AI’s machine learning platform enabled PayPal to strengthen fraud detection capabilitiesRETAIL & ECOMMERCE- Case Study 1: California Design Den partnered with Google Cloud Platform to implement machine learning solutionsIT/ITES- Case Study 1: Contentree helped Consensus simplify data wrangling process and make it efficient- Case Study 2: DataRobot’s automated machine learning platform helped Demyst automate data science processesHEALTHCARE & LIFESCIENCES- Case Study 1: DataRobot helped Evariant automate patient risk stratification and readmission predictionMEDIA & ENTERTAINMENT- Case Study 1: Meredith Corporation worked with Google Cloud to build data analytics platform to handle large volumes of dataTRANSPORTATION & LOGISTICS- Case Study 1: DMWay enabled PGL to integrate and analyze data from multiple sourcesENERGY & UTILITIES- Case Study 1: SparkCognition helped oil & gas industry to build predictive models by leveraging automated machine learning solutions
-
5.4 ECOSYSTEM ANALYSIS
- 5.5 HISTORY OF AUTOMATED MACHINE LEARNING
- 5.6 AUTOMATED MACHINE LEARNING PIPELINE FRAMEWORK
-
5.7 VALUE CHAIN ANALYSISDATA COLLECTION & PREPARATIONALGORITHM DEVELOPMENTMODEL TRAININGMODEL TESTING AND VALIDATIONDEPLOYMENT AND INTEGRATIONMAINTENANCE AND SUPPORT
- 5.8 PRICING MODEL ANALYSIS
-
5.9 PATENT ANALYSISMETHODOLOGYDOCUMENT TYPEINNOVATION AND PATENT APPLICATIONS- Top applicants
-
5.10 AUTOMATED MACHINE LEARNING TECHNIQUESBAYESIAN OPTIMIZATIONREINFORCEMENT LEARNINGEVOLUTIONARY ALGORITHMGRADIENT APPROACHES
- 5.11 COMPARISON OF AUTOAI AND AUTOML SOLUTIONS
-
5.12 BUSINESS MODELS OF AUTOMLAPI MODELSAS-A-SERVICE MODELCLOUD MODEL
-
5.13 TECHNOLOGY ANALYSISRELATED TECHNOLOGIES- Supervised learning- Unsupervised learning- Natural language processing- Computer vision- Transfer learningALLIED TECHNOLOGIES- Cloud computing- Robotics- Federated learning
-
5.14 PORTER’S FIVE FORCES ANALYSISTHREAT FROM NEW ENTRANTSTHREAT FROM SUBSTITUTESBARGAINING POWER OF SUPPLIERSBARGAINING POWER OF BUYERSINTENSITY OF COMPETITIVE RIVALRY
- 5.15 KEY CONFERENCES & EVENTS
-
5.16 REGULATORY LANDSCAPEREGULATORY BODIES, GOVERNMENT AGENCIES, AND OTHER ORGANIZATIONS- North America- Europe- Asia Pacific- Middle East & Africa- Latin America
-
5.17 KEY STAKEHOLDERS & BUYING CRITERIAKEY STAKEHOLDERS IN BUYING PROCESSBUYING CRITERIA
- 5.18 BEST PRACTICES IN AUTOMATED MACHINE LEARNING MARKET
- 5.19 DISRUPTIONS IMPACTING BUYERS/CLIENTS IN AUTOMATED MACHINE LEARNING MARKET
- 5.20 FUTURE DIRECTIONS OF AUTOMATED MACHINE LEARNING LANDSCAPE
-
6.1 INTRODUCTIONOFFERINGS: MARKET DRIVERS
-
6.2 SOLUTIONSAUTOMATED MACHINE LEARNING SOLUTIONS, BY TYPE- Platforms- SoftwareAUTOMATED MACHINE LEARNING SOLUTIONS, BY DEPLOYMENT- On-premises- Cloud
-
6.3 SERVICESCONSULTING SERVICES- Rising demand for expert guidance on machine learning strategies to drive growth of automated machine learning consulting servicesDEPLOYMENT AND INTEGRATION- Rising demand for integrating machine learning models into existing workflows and applications to boost adoption of AutoML deployment and integration servicesTRAINING, SUPPORT, AND MAINTENANCE- Rising preference for optimal model performance and accuracy to drive use of AutoML training, support, and maintenance services
-
7.1 INTRODUCTIONAPPLICATIONS: MARKET DRIVERS
-
7.2 DATA PROCESSINGGROWING NEED TO DETECT AND CORRECT DATA ERRORS TO DRIVE ADOPTION OF AUTOML SOLUTIONS FOR DATA PROCESSINGCLEANINGTRANSFORMATIONVISUALIZATION
-
7.3 MODEL SELECTIONRISING DEMAND FOR AUTOMATED TECHNIQUES TO HANDLE COMPLEX DATA TO BOOST GROWTH OF AUTOML SOLUTIONS FOR MODEL SELECTIONSCALINGMONITORINGVERSIONING
-
7.4 HYPERPARAMETER OPTIMIZATION & TUNINGINCREASED ADOPTION OF AUTOML ALGORITHMS FOR HYPERPARAMETER OPTIMIZATION TO DRIVE MARKET GROWTHGRID SEARCHRANDOM SEARCHBAYESIAN SEARCH
-
7.5 FEATURE ENGINEERINGRISING NEED TO TRANSFORM RAW DATA INTO SET OF FEATURES FOR USE IN MACHINE LEARNING MODELS TO BOOST ADOPTION OF AUTOML SOLUTIONS IN FEATURE ENGINEERING
-
7.6 MODEL ENSEMBLINGGROWING IMPORTANCE OF IMPROVING PREDICTION ACCURACY TO PROPEL GROWTH OF AUTOML SOLUTIONS FOR MODEL ENSEMBLINGINFRASTRUCTURE & FORMATINTEGRATIONMAINTENANCE
- 7.7 OTHER APPLICATIONS
-
8.1 INTRODUCTIONVERTICALS: MARKET DRIVERS
-
8.2 BANKING, FINANCIAL SERVICES, AND INSURANCENEED TO OPTIMIZE BUSINESS PERFORMANCE WITH REAL-TIME ANALYTICS TO DRIVE USE OF AUTOML SOLUTIONS IN BFSI SECTORCREDIT SCORINGFRAUD DETECTIONRISK ANALYSIS & MANAGEMENTOTHER BFSI SUB-VERTICALS
-
8.3 HEALTHCARE & LIFE SCIENCESDEMAND FOR IMPROVED DIAGNOSES AND PERSONALIZED TREATMENT PLANS TO DRIVE MARKET FOR AI AND ML SOLUTIONS FOR HEALTHCARE & LIFE SCIENCES INDUSTRYANOMALY DETECTIONDISEASE DIAGNOSISDRUG DISCOVERYOTHER HEALTHCARE SUB-VERTICALS
-
8.4 RETAIL & ECOMMERCEGROWING NEED FOR PERSONALIZATION AND OPTIMIZATION IN HIGHLY COMPETITIVE INDUSTRIES TO BOOST MARKET GROWTHDEMAND FORECASTINGPRICE OPTIMIZATIONRECOMMENDATION ENGINESSENTIMENT ANALYSISSOCIAL MEDIA ANALYTICSCHATBOTS FOR CUSTOMER SERVICE & SUPPORTOTHER RETAIL & ECOMMERCE SUB-VERTICALS
-
8.5 MANUFACTURINGAUTOML SOLUTIONS TO OPTIMIZE MANUFACTURING PROCESS AND IMPROVE EFFICIENCYPREDICTIVE MAINTENANCEQUALITY CONTROLROBOTIC PROCESS AUTOMATIONSUPPLY CHAIN OPTIMIZATIONOTHER MANUFACTURING SUB-VERTICALS
-
8.6 GOVERNMENT & DEFENSERISING NEED TO EMPOWER NATIONAL SECURITY AND PUBLIC SERVICES TO DRIVE ADOPTION OF AUTOML PLATFORMS IN GOVERNMENT & DEFENSE SECTORCYBERSECURITY THREAT DETECTIONFRAUD DETECTION & PREVENTIONNATURAL DISASTER MANAGEMENTCUSTOMER SERVICE CHATBOTSOTHER GOVERNMENT & DEFENSE SUB-VERTICALS
-
8.7 TELECOMMUNICATIONSNEED FOR ENHANCED CUSTOMER SERVICE TO BOOST USE OF AUTOML SOLUTIONS IN TELECOMMUNICATIONS INDUSTRYCYBERSECURITY THREAT DETECTIONNETWORK OPTIMIZATIONPREDICTIVE MAINTENANCEFRAUD DETECTION & PREVENTIONCHATBOTS & VIRTUAL ASSISTANCEOTHER TELECOMMUNICATIONS SUB-VERTICALS
-
8.8 IT/ITESNEED TO OPTIMIZE PROCESSES AND ENHANCE CYBERSECURITY TO PROPEL GROWTH OF AUTOMATED MACHINE LEARNING MARKET FOR IT/ITES SECTORPREDICTIVE MAINTENANCEVIRTUAL ASSISTANTS FOR CUSTOMER SUPPORTNETWORK OPTIMIZATIONOTHER IT/ITES SUB-VERTICALS
-
8.9 AUTOMOTIVE, TRANSPORTATION, AND LOGISTICSAUTOMATED MACHINE LEARNING SOLUTIONS TO ENABLE ORGANIZATIONS TO LEVERAGE DATA AND GAIN INSIGHTS FOR BETTER BUSINESS DECISIONSAUTONOMOUS VEHICLESROUTE OPTIMIZATIONFUEL EFFICIENCY PREDICTION & OPTIMIZATIONHUMAN MACHINE INTERFACE (HMI)SEMI-AUTONOMOUS DRIVINGROBOTIC PROCESS AUTOMATIONOTHER AUTOMOTIVE, TRANSPORTATION, AND LOGISTICS SUB-VERTICALS
-
8.10 MEDIA & ENTERTAINMENTUSE OF AUTOML SOLUTIONS TO ENSURE IMPROVED CONTENT DISCOVERYIMAGE & SPEECH RECOGNITIONRECOMMENDATION SYSTEMSSENTIMENT ANALYSISOTHER MEDIA & ENTERTAINMENT SUB-VERTICALS
- 8.11 OTHER VERTICALS
- 9.1 INTRODUCTION
-
9.2 NORTH AMERICANORTH AMERICA: MARKET DRIVERSNORTH AMERICA: RECESSION IMPACTUS- Growing demand for efficient ways to build and deploy machine learning models to drive market growthCANADA- Rising adoption of machine learning applications in various industries across Canada to fuel market growth
-
9.3 EUROPEEUROPE: MARKET DRIVERSEUROPE: RECESSION IMPACTGERMANY- Strong IT infrastructure and robust regulatory framework to drive AutoML market in GermanyFRANCE- Country’s thriving startup ecosystem to boost adoption of automated machine learning solutionsITALY- Significant initiatives taken by government to promote use of automated machine learning platforms to boost market growthSPAIN- Rising technological investments by major players to boost popularity of AutoML platforms and solutions in SpainNORDIC- Increasing research and development in AI and machine learning in Nordic countries to drive market growthREST OF EUROPE
-
9.4 ASIA PACIFICASIA PACIFIC: MARKET DRIVERSASIA PACIFIC: RECESSION IMPACTCHINA- Heavy investments made in machine learning technology to drive growth of automated machine learning solutions in ChinaJAPAN- Growing need for technological enhancements to boost growth of AutoML solutions and services in JapanSOUTH KOREA- Strong focus on developing cutting-edge technologies to boost use of AutoML solutions across sectors in South KoreaASEAN- Rising demand to leverage machine learning solutions for competitive advantage to boost growth of marketAUSTRALIA & NEW ZEALAND- Increased innovations by major companies specializing in machine learning to drive adoption of AutoML solutions across industriesREST OF ASIA PACIFIC
-
9.5 MIDDLE EAST & AFRICAMIDDLE EAST & AFRICA: MARKET DRIVERSMIDDLE EAST & AFRICA: RECESSION IMPACTSAUDI ARABIA- Saudi Arabia’s commitment to leveraging AI and ML technologies to drive market growthUAE- Rising growth of advanced technologies to drive market for AI and ML solutions and servicesISRAEL- Growing investments in AI and ML research by major players to boost growth of automated machine learning market in IsraelTURKEY- Growing ecosystem and adoption of machine learning technology across industries to boost market growth in TurkeySOUTH AFRICA- Increasing investments and initiatives from governments and private sector to drive popularity of AI and ML solutionsREST OF MIDDLE EAST & AFRICA
-
9.6 LATIN AMERICALATIN AMERICA: MARKET DRIVERSLATIN AMERICA: RECESSION IMPACTBRAZIL- Significant government support to drive adoption of AI and ML technologies across industriesMEXICO- Rapid growth in country’s technology sector to drive market for automated machine learningARGENTINA- Government incentives to foreign companies for investments in country’s technology sector to boost AutoML market growthREST OF LATIN AMERICA
- 10.1 OVERVIEW
- 10.2 STRATEGIES ADOPTED BY KEY PLAYERS
- 10.3 REVENUE ANALYSIS
- 10.4 MARKET SHARE ANALYSIS
-
10.5 EVALUATION QUADRANT MATRIX FOR KEY PLAYERSSTARSEMERGING LEADERSPERVASIVE PLAYERSPARTICIPANTS
-
10.6 EVALUATION QUADRANT MATRIX FOR SMES/STARTUPSPROGRESSIVE COMPANIESRESPONSIVE COMPANIESDYNAMIC COMPANIESSTARTING BLOCKS
- 10.7 COMPETITIVE BENCHMARKING
-
10.8 AUTOMATED MACHINE LEARNING PRODUCT LANDSCAPECOMPARATIVE ANALYSIS OF AUTOMATED MACHINE LEARNING PRODUCTS
-
10.9 COMPETITIVE SCENARIOPRODUCT LAUNCHESDEALSOTHERS
- 10.10 VALUATION AND FINANCIAL METRICS OF KEY AUTOMATED MACHINE LEARNING VENDORS
- 10.11 YTD PRICE TOTAL RETURN AND STOCK BETA OF KEY AUTOMATED MACHINE LEARNING VENDORS
- 11.1 INTRODUCTION
-
11.2 KEY PLAYERSIBM- Business overview- Products/Solutions offered- Recent developments- MnM viewORACLE- Business overview- Products/Solutions offered- Recent developments- MnM viewMICROSOFT- Business overview- Products/Solutions offered- Recent developments- MnM viewSERVICENOW- Business overview- Products/Solutions offered- Recent developments- MnM viewGOOGLE- Business overview- Products/Solutions offered- Recent developments- MnM viewBAIDU- Business overview- Products offered- Recent developmentsAWS- Business overview- Products/Services offered- Recent developmentsALTERYX- Business overview- Products offered- Recent developmentsHPE- Business overview- Products/Solutions offered- Recent developmentsSALESFORCE- Business overview- Products/Solutions offered- Recent developmentsALTAIR- Business overview- Products/Solutions offered- Recent developmentsTERADATA- Business overview- Products/Solutions offeredH2O.AI- Business overview- Products/Solutions offered- Recent developmentsDATAROBOT- Business overview- Products/Services offeredBIGML- Business overview- Products/Solutions offered- Recent developmentsDATABRICKS- Business overview- Products/Solutions offered- Recent developmentsDATAIKU- Business overview- Products/Solutions offered- Recent developmentsMATHWORKS- Business overview- Products/Solutions offered- Recent developmentsSPARKCOGNITION- Business overview- Products/Solutions offered- Recent developmentsQLIK- Business overview- Products/Solutions offered- Recent developments
-
11.3 OTHER PLAYERSALIBABA CLOUDAPPIERSQUARKAIBLEDATAFOLDBOOST.AITAZI AIAKKIOVALOHAIDOTDATA
-
12.1 GENERATIVE AI MARKETMARKET DEFINITIONMARKET OVERVIEWGENERATIVE AI MARKET, BY OFFERINGGENERATIVE AI MARKET, BY APPLICATIONGENERATIVE AI MARKET, BY VERTICALGENERATIVE AI MARKET, BY REGION
-
12.2 ARTIFICIAL INTELLIGENCE MARKETMARKET DEFINITIONMARKET OVERVIEWARTIFICIAL INTELLIGENCE MARKET, BY OFFERINGARTIFICIAL INTELLIGENCE MARKET, BY TECHNOLOGYARTIFICIAL INTELLIGENCE MARKET, BY DEPLOYMENT MODEARTIFICIAL INTELLIGENCE MARKET, BY ORGANIZATION SIZEARTIFICIAL INTELLIGENCE MARKET, BY BUSINESS FUNCTIONARTIFICIAL INTELLIGENCE MARKET, BY VERTICALARTIFICIAL INTELLIGENCE MARKET, BY REGION
- 13.1 DISCUSSION GUIDE
- 13.2 KNOWLEDGESTORE: MARKETSANDMARKETS’ SUBSCRIPTION PORTAL
- 13.3 CUSTOMIZATION OPTIONS
- 13.4 RELATED REPORTS
- 13.5 AUTHOR DETAILS
- TABLE 1 USD EXCHANGE RATES, 2020–2022
- TABLE 2 FACTOR ANALYSIS
- TABLE 3 IMPACT OF RECESSION ON GLOBAL AUTOMATED MACHINE LEARNING MARKET
- TABLE 4 GLOBAL MARKET SIZE AND GROWTH RATE, 2017–2022 (USD MILLION, Y-O-Y%)
- TABLE 5 GLOBAL AUTOMATED MACHINE LEARNING MARKET SIZE AND GROWTH RATE, 2023–2028 (USD MILLION, Y-O-Y%)
- TABLE 6 MARKET: PLATFORM PROVIDERS
- TABLE 7 MARKET: SERVICE PROVIDERS
- TABLE 8 MARKET: TECHNOLOGY PROVIDERS
- TABLE 9 MARKET: REGULATORY BODIES
- TABLE 10 AUTOMATED MACHINE LEARNING PIPELINE FRAMEWORK
- TABLE 11 AUTOMATED MACHINE LEARNING MARKET: PRICING LEVELS
- TABLE 12 PATENTS FILED, 2018–2021
- TABLE 13 TOP 20 PATENT OWNERS, 2018–2021
- TABLE 14 LIST OF PATENTS IN AUTOMATED MACHINE LEARNING MARKET, 2021–2023
- TABLE 15 COMPARISON BETWEEN AUTOAI AND AUTOML SOLUTIONS
- TABLE 16 PORTER’S FIVE FORCES ANALYSIS
- TABLE 17 DETAILED LIST OF CONFERENCES & EVENTS, 2023–2024
- TABLE 18 NORTH AMERICA: REGULATORY BODIES, GOVERNMENT AGENCIES, AND OTHER ORGANIZATIONS
- TABLE 19 EUROPE: REGULATORY BODIES, GOVERNMENT AGENCIES, AND OTHER ORGANIZATIONS
- TABLE 20 ASIA PACIFIC: LIST OF REGULATORY BODIES, GOVERNMENT AGENCIES, AND OTHER ORGANIZATIONS
- TABLE 21 ROW: REGULATORY BODIES, GOVERNMENT AGENCIES, AND OTHER ORGANIZATIONS
- TABLE 22 INFLUENCE OF STAKEHOLDERS ON BUYING PROCESS FOR TOP THREE VERTICALS
- TABLE 23 KEY BUYING CRITERIA FOR TOP THREE VERTICALS
- TABLE 24 SHORT-TERM ROADMAP, 2023–2025
- TABLE 25 MID-TERM ROADMAP, 2026–2028
- TABLE 26 LONG-TERM ROADMAP, 2029–2030
- TABLE 27 AUTOMATED MACHINE LEARNING MARKET, BY OFFERING, 2017–2022 (USD MILLION)
- TABLE 28 MARKET, BY OFFERING, 2023–2028 (USD MILLION)
- TABLE 29 SOLUTIONS: MARKET, BY REGION, 2017–2022 (USD MILLION)
- TABLE 30 SOLUTIONS: MARKET, BY REGION, 2023–2028 (USD MILLION)
- TABLE 31 SOLUTIONS: MARKET, BY TYPE, 2017–2022 (USD MILLION)
- TABLE 32 SOLUTIONS: MARKET, BY TYPE, 2023–2028 (USD MILLION)
- TABLE 33 PLATFORMS: MARKET, BY REGION, 2017–2022 (USD MILLION)
- TABLE 34 PLATFORMS: MARKET, BY REGION, 2023–2028 (USD MILLION)
- TABLE 35 SOFTWARE: MARKET, BY REGION, 2017–2022 (USD MILLION)
- TABLE 36 SOFTWARE: MARKET, BY REGION, 2023–2028 (USD MILLION)
- TABLE 37 SOLUTIONS: MARKET, BY DEPLOYMENT, 2017–2022 (USD MILLION)
- TABLE 38 SOLUTIONS: MARKET, BY DEPLOYMENT, 2023–2028 (USD MILLION)
- TABLE 39 ON-PREMISES: MARKET, BY REGION, 2017–2022 (USD MILLION)
- TABLE 40 ON-PREMISES: MARKET, BY REGION, 2023–2028 (USD MILLION)
- TABLE 41 CLOUD: MARKET, BY REGION, 2017–2022 (USD MILLION)
- TABLE 42 CLOUD: MARKET, BY REGION, 2023–2028 (USD MILLION)
- TABLE 43 SERVICES: MARKET, BY TYPE, 2017–2022 (USD MILLION)
- TABLE 44 SERVICES: MARKET, BY TYPE, 2023–2028 (USD MILLION)
- TABLE 45 SERVICES: MARKET, BY REGION, 2017–2022 (USD MILLION)
- TABLE 46 SERVICES: MARKET, BY REGION, 2023–2028 (USD MILLION)
- TABLE 47 CONSULTING SERVICES: MARKET, BY REGION, 2017–2022 (USD MILLION)
- TABLE 48 CONSULTING SERVICES: MARKET, BY REGION, 2023–2028 (USD MILLION)
- TABLE 49 DEPLOYMENT AND INTEGRATION: MARKET, BY REGION, 2017–2022 (USD MILLION)
- TABLE 50 DEPLOYMENT AND INTEGRATION: MARKET, BY REGION, 2023–2028 (USD MILLION)
- TABLE 51 TRAINING, SUPPORT, AND MAINTENANCE: MARKET, BY REGION, 2017–2022 (USD MILLION)
- TABLE 52 TRAINING, SUPPORT, AND MAINTENANCE: MARKET, BY REGION, 2023–2028 (USD MILLION)
- TABLE 53 AUTOMATED MACHINE LEARNING MARKET, BY APPLICATION, 2017–2022 (USD MILLION)
- TABLE 54 MARKET, BY APPLICATION, 2023–2028 (USD MILLION)
- TABLE 55 DATA PROCESSING: MARKET, BY REGION, 2017–2022 (USD MILLION)
- TABLE 56 DATA PROCESSING: MARKET, BY REGION, 2023–2028 (USD MILLION)
- TABLE 57 MODEL SELECTION: MARKET, BY REGION, 2017–2022 (USD MILLION)
- TABLE 58 MODEL SELECTION: MARKET, BY REGION, 2023–2028 (USD MILLION)
- TABLE 59 HYPERPARAMETER TUNING & OPTIMIZATION: MARKET, BY REGION, 2017–2022 (USD MILLION)
- TABLE 60 HYPERPARAMETER TUNING & OPTIMIZATION: MARKET, BY REGION, 2023–2028 (USD MILLION)
- TABLE 61 FEATURE ENGINEERING: MARKET, BY REGION, 2017–2022 (USD MILLION)
- TABLE 62 FEATURE ENGINEERING: MARKET, BY REGION, 2023–2028 (USD MILLION)
- TABLE 65 OTHER APPLICATIONS: MARKET, BY REGION, 2017–2022 (USD MILLION)
- TABLE 66 OTHER APPLICATIONS: MARKET, BY REGION, 2023–2028 (USD MILLION)
- TABLE 67 AUTOMATED MACHINE LEARNING MARKET, BY VERTICAL, 2017–2022 (USD MILLION)
- TABLE 68 MARKET, BY VERTICAL, 2023–2028 (USD MILLION)
- TABLE 69 BFSI: USE CASES
- TABLE 70 BFSI: MARKET, BY REGION, 2017–2022 (USD MILLION)
- TABLE 71 BFSI: MARKET, BY REGION, 2023–2028 (USD MILLION)
- TABLE 72 BFSI: MARKET, BY SUB-VERTICAL, 2017–2022 (USD MILLION)
- TABLE 73 BFSI: MARKET, BY SUB-VERTICAL, 2023–2028 (USD MILLION)
- TABLE 74 HEALTHCARE & LIFESCIENCES: USE CASES
- TABLE 75 HEALTHCARE & LIFE SCIENCES: MARKET, BY REGION, 2017–2022 (USD MILLION)
- TABLE 76 HEALTHCARE & LIFE SCIENCES: MARKET, BY REGION, 2023–2028 (USD MILLION)
- TABLE 77 HEALTHCARE & LIFE SCIENCES: MARKET, BY SUB-VERTICAL, 2017–2022 (USD MILLION)
- TABLE 78 HEALTHCARE & LIFE SCIENCES: MARKET, BY SUB-VERTICAL, 2023–2028 (USD MILLION)
- TABLE 79 RETAIL & ECOMMERCE: USE CASES
- TABLE 80 RETAIL & ECOMMERCE: MARKET, BY REGION, 2017–2022 (USD MILLION)
- TABLE 81 RETAIL & ECOMMERCE: MARKET, BY REGION, 2023–2028 (USD MILLION)
- TABLE 82 RETAIL & ECOMMERCE: MARKET, BY SUB-VERTICAL, 2017–2022 (USD MILLION)
- TABLE 83 RETAIL & ECOMMERCE: MARKET, BY SUB-VERTICAL, 2023–2028 (USD MILLION)
- TABLE 84 MANUFACTURING: USE CASES
- TABLE 85 MANUFACTURING: MARKET, BY REGION, 2017–2022 (USD MILLION)
- TABLE 86 MANUFACTURING: AUTOMATED MACHINE LEARNING MARKET, BY REGION, 2023–2028 (USD MILLION)
- TABLE 87 MANUFACTURING: MARKET, BY SUB-VERTICAL, 2017–2022 (USD MILLION)
- TABLE 88 MANUFACTURING: MARKET, BY SUB-VERTICAL, 2023–2028 (USD MILLION)
- TABLE 89 GOVERNMENT & DEFENSE: USE CASES
- TABLE 90 GOVERNMENT & DEFENSE: MARKET, BY REGION, 2017–2022 (USD MILLION)
- TABLE 91 GOVERNMENT & DEFENSE: MARKET, BY REGION, 2023–2028 (USD MILLION)
- TABLE 92 GOVERNMENT & DEFENSE: MARKET, BY SUB-VERTICAL, 2017–2022 (USD MILLION)
- TABLE 93 GOVERNMENT & DEFENSE: MARKET, BY SUB-VERTICAL, 2023–2028 (USD MILLION)
- TABLE 94 TELECOMMUNICATIONS: USE CASES
- TABLE 95 TELECOMMUNICATIONS: AUTOMATED MACHINE LEARNING MARKET, BY REGION, 2017–2022 (USD MILLION)
- TABLE 96 TELECOMMUNICATIONS: MARKET, BY REGION, 2023–2028 (USD MILLION)
- TABLE 97 TELECOMMUNICATIONS: MARKET, BY SUB-VERTICAL, 2017–2022 (USD MILLION)
- TABLE 98 TELECOMMUNICATIONS: MARKET, BY SUB-VERTICAL, 2023–2028 (USD MILLION)
- TABLE 99 IT/ITES: USE CASES
- TABLE 100 IT/ITES: MARKET, BY REGION, 2017–2022 (USD MILLION)
- TABLE 101 IT/ITES: MARKET, BY REGION, 2023–2028 (USD MILLION)
- TABLE 102 IT/ITES: MARKET, BY SUB-VERTICAL, 2017–2022 (USD MILLION)
- TABLE 103 IT/ITES: MARKET, BY SUB-VERTICAL, 2023–2028 (USD MILLION)
- TABLE 104 AUTOMOTIVE, TRANSPORTATION, AND LOGISTICS: USE CASES
- TABLE 105 AUTOMOTIVE, TRANSPORTATION, AND LOGISTICS: MARKET, BY REGION, 2017–2022 (USD MILLION)
- TABLE 106 AUTOMOTIVE, TRANSPORTATION, AND LOGISTICS: MARKET, BY REGION, 2023–2028 (USD MILLION)
- TABLE 107 AUTOMOTIVE, TRANSPORTATION, AND LOGISTICS: MARKET, BY SUB-VERTICAL, 2017–2022 (USD MILLION)
- TABLE 108 AUTOMOTIVE, TRANSPORTATION, AND LOGISTICS: MARKET, BY SUB-VERTICAL, 2023–2028 (USD MILLION)
- TABLE 109 MEDIA & ENTERTAINMENT: USE CASES
- TABLE 110 MEDIA & ENTERTAINMENT: MARKET, BY REGION, 2017–2022 (USD MILLION)
- TABLE 111 MEDIA & ENTERTAINMENT: AUTOMATED MACHINE LEARNING MARKET, BY REGION, 2023–2028 (USD MILLION)
- TABLE 112 MEDIA & ENTERTAINMENT: MARKET, BY SUB-VERTICAL, 2017–2022 (USD MILLION)
- TABLE 113 MEDIA & ENTERTAINMENT: MARKET, BY SUB-VERTICAL, 2023–2028 (USD MILLION)
- TABLE 114 OTHER VERTICALS: MARKET, BY REGION, 2017–2022 (USD MILLION)
- TABLE 115 OTHER VERTICALS: MARKET, BY REGION, 2023–2028 (USD MILLION)
- TABLE 116 MARKET, BY REGION, 2017–2022 (USD MILLION)
- TABLE 117 MARKET, BY REGION, 2023–2028 (USD MILLION)
- TABLE 118 NORTH AMERICA: MARKET, BY OFFERING, 2017–2022 (USD MILLION)
- TABLE 119 NORTH AMERICA: MARKET, BY OFFERING, 2023–2028 (USD MILLION)
- TABLE 120 NORTH AMERICA: MARKET, BY TYPE, 2017–2022 (USD MILLION)
- TABLE 121 NORTH AMERICA: MARKET, BY TYPE, 2023–2028 (USD MILLION)
- TABLE 122 NORTH AMERICA: AUTOMATED MACHINE LEARNING MARKET, BY DEPLOYMENT, 2017–2022 (USD MILLION)
- TABLE 123 NORTH AMERICA: MARKET, BY DEPLOYMENT, 2023–2028 (USD MILLION)
- TABLE 124 NORTH AMERICA: MARKET, BY SERVICE, 2017–2022 (USD MILLION)
- TABLE 125 NORTH AMERICA: MARKET, BY SERVICE, 2023–2028 (USD MILLION)
- TABLE 126 NORTH AMERICA: MARKET, BY APPLICATION, 2017–2022 (USD MILLION)
- TABLE 127 NORTH AMERICA: MARKET, BY APPLICATION, 2023–2028 (USD MILLION)
- TABLE 128 NORTH AMERICA: MARKET, BY VERTICAL, 2017–2022 (USD MILLION)
- TABLE 129 NORTH AMERICA: MARKET, BY VERTICAL, 2023–2028 (USD MILLION)
- TABLE 130 NORTH AMERICA: MARKET, BY COUNTRY, 2017–2022 (USD MILLION)
- TABLE 131 NORTH AMERICA: MARKET, BY COUNTRY, 2023–2028 (USD MILLION)
- TABLE 132 US: MARKET, BY OFFERING, 2017–2022 (USD MILLION)
- TABLE 133 US: MARKET, BY OFFERING, 2023–2028 (USD MILLION)
- TABLE 134 US: MARKET, BY TYPE, 2017–2022 (USD MILLION)
- TABLE 135 US: MARKET, BY TYPE, 2023–2028 (USD MILLION)
- TABLE 136 US: MARKET, BY DEPLOYMENT, 2017–2022 (USD MILLION)
- TABLE 137 US: MARKET, BY DEPLOYMENT, 2023–2028 (USD MILLION)
- TABLE 138 US: MARKET, BY SERVICE, 2017–2022 (USD MILLION)
- TABLE 139 US: MARKET, BY SERVICE, 2023–2028 (USD MILLION)
- TABLE 140 EUROPE: MARKET, BY OFFERING, 2017–2022 (USD MILLION)
- TABLE 141 EUROPE: AUTOMATED MACHINE LEARNING MARKET, BY OFFERING, 2023–2028 (USD MILLION)
- TABLE 142 EUROPE: MARKET, BY TYPE, 2017–2022 (USD MILLION)
- TABLE 143 EUROPE: MARKET, BY TYPE, 2023–2028 (USD MILLION)
- TABLE 144 EUROPE: MARKET, BY DEPLOYMENT, 2017–2022 (USD MILLION)
- TABLE 145 EUROPE: MARKET, BY DEPLOYMENT, 2023–2028 (USD MILLION)
- TABLE 146 EUROPE: MARKET, BY SERVICE, 2017–2022 (USD MILLION)
- TABLE 147 EUROPE: MARKET, BY SERVICE, 2023–2028 (USD MILLION)
- TABLE 148 EUROPE: MARKET, BY APPLICATION, 2017–2022 (USD MILLION)
- TABLE 149 EUROPE: MARKET, BY APPLICATION, 2023–2028 (USD MILLION)
- TABLE 150 EUROPE: MARKET, BY VERTICAL, 2017–2022 (USD MILLION)
- TABLE 151 EUROPE: MARKET, BY VERTICAL, 2023–2028 (USD MILLION)
- TABLE 152 EUROPE: MARKET, BY COUNTRY, 2017–2022 (USD MILLION)
- TABLE 153 EUROPE: MARKET, BY COUNTRY, 2023–2028 (USD MILLION)
- TABLE 154 UK: MARKET, BY OFFERING, 2017–2022 (USD MILLION)
- TABLE 155 UK: MARKET, BY OFFERING, 2023–2028 (USD MILLION)
- TABLE 156 UK: MARKET, BY TYPE, 2017–2022 (USD MILLION)
- TABLE 157 UK: MARKET, BY TYPE, 2023–2028 (USD MILLION)
- TABLE 158 UK: MARKET, BY DEPLOYMENT, 2017–2022 (USD MILLION)
- TABLE 159 UK: MARKET, BY DEPLOYMENT, 2023–2028 (USD MILLION)
- TABLE 160 UK: MARKET, BY SERVICE, 2017–2022 (USD MILLION)
- TABLE 161 UK: MARKET, BY SERVICE, 2023–2028 (USD MILLION)
- TABLE 162 ASIA PACIFIC: AUTOMATED MACHINE LEARNING MARKET, BY OFFERING, 2017–2022 (USD MILLION)
- TABLE 163 ASIA PACIFIC: MARKET, BY OFFERING, 2023–2028 (USD MILLION)
- TABLE 164 ASIA PACIFIC: MARKET, BY TYPE, 2017–2022 (USD MILLION)
- TABLE 165 ASIA PACIFIC: MARKET, BY TYPE, 2023–2028 (USD MILLION)
- TABLE 166 ASIA PACIFIC: MARKET, BY DEPLOYMENT, 2017–2022 (USD MILLION)
- TABLE 167 ASIA PACIFIC: MARKET, BY DEPLOYMENT, 2023–2028 (USD MILLION)
- TABLE 168 ASIA PACIFIC: MARKET, BY SERVICE, 2017–2022 (USD MILLION)
- TABLE 169 ASIA PACIFIC: MARKET, BY SERVICE, 2023–2028 (USD MILLION)
- TABLE 170 ASIA PACIFIC: MARKET, BY APPLICATION, 2017–2022 (USD MILLION)
- TABLE 171 ASIA PACIFIC: MARKET, BY APPLICATION, 2023–2028 (USD MILLION)
- TABLE 172 ASIA PACIFIC: MARKET, BY VERTICAL, 2017–2022 (USD MILLION)
- TABLE 173 ASIA PACIFIC: MARKET, BY VERTICAL, 2023–2028 (USD MILLION)
- TABLE 174 ASIA PACIFIC: MARKET, BY COUNTRY, 2017–2022 (USD MILLION)
- TABLE 175 ASIA PACIFIC: MARKET, BY COUNTRY, 2023–2028 (USD MILLION)
- TABLE 176 CHINA: MARKET, BY OFFERING, 2017–2022 (USD MILLION)
- TABLE 177 CHINA: MARKET, BY OFFERING, 2023–2028 (USD MILLION)
- TABLE 178 CHINA: MARKET, BY TYPE, 2017–2022 (USD MILLION)
- TABLE 179 CHINA: MARKET, BY TYPE, 2023–2028 (USD MILLION)
- TABLE 180 CHINA: MARKET, BY DEPLOYMENT, 2017–2022 (USD MILLION)
- TABLE 181 CHINA: MARKET, BY DEPLOYMENT, 2023–2028 (USD MILLION)
- TABLE 182 CHINA: MARKET, BY SERVICE, 2017–2022 (USD MILLION)
- TABLE 183 CHINA: MARKET, BY SERVICE, 2023–2028 (USD MILLION)
- TABLE 184 MIDDLE EAST & AFRICA: MARKET, BY OFFERING, 2017–2022 (USD MILLION)
- TABLE 185 MIDDLE EAST & AFRICA: MARKET, BY OFFERING, 2023–2028 (USD MILLION)
- TABLE 186 MIDDLE EAST & AFRICA: AUTOMATED MACHINE LEARNING MARKET, BY TYPE, 2017–2022 (USD MILLION)
- TABLE 187 MIDDLE EAST & AFRICA: MARKET, BY TYPE, 2023–2028 (USD MILLION)
- TABLE 188 MIDDLE EAST & AFRICA: MARKET, BY DEPLOYMENT, 2017–2022 (USD MILLION)
- TABLE 189 MIDDLE EAST & AFRICA: MARKET, BY DEPLOYMENT, 2023–2028 (USD MILLION)
- TABLE 190 MIDDLE EAST & AFRICA: MARKET, BY SERVICE, 2017–2022 (USD MILLION)
- TABLE 191 MIDDLE EAST & AFRICA: MARKET, BY SERVICE, 2023–2028 (USD MILLION)
- TABLE 192 MIDDLE EAST & AFRICA: MARKET, BY APPLICATION, 2017–2022 (USD MILLION)
- TABLE 193 MIDDLE EAST & AFRICA: MARKET, BY APPLICATION, 2023–2028 (USD MILLION)
- TABLE 194 MIDDLE EAST & AFRICA: MARKET, BY VERTICAL, 2017–2022 (USD MILLION)
- TABLE 195 MIDDLE EAST & AFRICA: MARKET, BY VERTICAL, 2023–2028 (USD MILLION)
- TABLE 196 MIDDLE EAST & AFRICA: MARKET, BY COUNTRY, 2017–2022 (USD MILLION)
- TABLE 197 MIDDLE EAST & AFRICA: MARKET, BY COUNTRY, 2023–2028 (USD MILLION)
- TABLE 198 LATIN AMERICA: MARKET, BY OFFERING, 2017–2022 (USD MILLION)
- TABLE 199 LATIN AMERICA: AUTOMATED MACHINE LEARNING MARKET, BY OFFERING, 2023–2028 (USD MILLION)
- TABLE 200 LATIN AMERICA: MARKET, BY TYPE, 2017–2022 (USD MILLION)
- TABLE 201 LATIN AMERICA: MARKET, BY TYPE, 2023–2028 (USD MILLION)
- TABLE 202 LATIN AMERICA: MARKET, BY DEPLOYMENT, 2017–2022 (USD MILLION)
- TABLE 203 LATIN AMERICA: MARKET, BY DEPLOYMENT, 2023–2028 (USD MILLION)
- TABLE 204 LATIN AMERICA: MARKET, BY SERVICE, 2017–2022 (USD MILLION)
- TABLE 205 LATIN AMERICA: MARKET, BY SERVICE, 2023–2028 (USD MILLION)
- TABLE 206 LATIN AMERICA: MARKET, BY APPLICATION, 2017–2022 (USD MILLION)
- TABLE 207 LATIN AMERICA: MARKET, BY APPLICATION, 2023–2028 (USD MILLION)
- TABLE 208 LATIN AMERICA: MARKET, BY VERTICAL, 2017–2022 (USD MILLION)
- TABLE 209 LATIN AMERICA: MARKET, BY VERTICAL, 2023–2028 (USD MILLION)
- TABLE 210 LATIN AMERICA: MARKET, BY COUNTRY, 2017–2022 (USD MILLION)
- TABLE 211 LATIN AMERICA: MARKET, BY COUNTRY, 2023–2028 (USD MILLION)
- TABLE 212 STRATEGIES ADOPTED BY KEY PLAYERS
- TABLE 213 AUTOMATED MACHINE LEARNING MARKET: INTENSITY OF COMPETITIVE RIVALRY
- TABLE 214 COMPETITIVE BENCHMARKING FOR KEY PLAYERS, 2023
- TABLE 215 DETAILED LIST OF KEY SMES/STARTUPS
- TABLE 216 COMPETITIVE BENCHMARKING FOR SMES/STARTUPS, 2023
- TABLE 217 COMPARATIVE ANALYSIS OF AUTOMATED MACHINE LEARNING PRODUCTS
- TABLE 218 AUTOMATED MACHINE LEARNING MARKET: PRODUCT LAUNCHES, 2020–2023
- TABLE 219 MARKET: DEALS, 2020–2023
- TABLE 220 AUTOMATED MACHINE LEARNING MARKET: OTHERS, 2020–2022
- TABLE 221 IBM: BUSINESS OVERVIEW
- TABLE 222 IBM: PRODUCTS/SOLUTIONS OFFERED
- TABLE 223 IBM: PRODUCT LAUNCHES
- TABLE 224 IBM: DEALS
- TABLE 225 ORACLE: BUSINESS OVERVIEW
- TABLE 226 ORACLE: PRODUCTS/SOLUTIONS OFFERED
- TABLE 227 ORACLE: PRODUCT LAUNCHES
- TABLE 228 ORACLE: DEALS
- TABLE 229 ORACLE: OTHERS
- TABLE 230 MICROSOFT: BUSINESS OVERVIEW
- TABLE 231 MICROSOFT: PRODUCTS/SOLUTIONS OFFERED
- TABLE 232 MICROSOFT: PRODUCT LAUNCHES
- TABLE 233 MICROSOFT: DEALS
- TABLE 234 SERVICENOW: BUSINESS OVERVIEW
- TABLE 235 SERVICENOW: PRODUCTS/SOLUTIONS OFFERED
- TABLE 236 SERVICENOW: PRODUCT LAUNCHES
- TABLE 237 SERVICENOW: DEALS
- TABLE 238 GOOGLE: BUSINESS OVERVIEW
- TABLE 239 GOOGLE: PRODUCTS/SOLUTIONS OFFERED
- TABLE 240 GOOGLE: PRODUCT LAUNCHES
- TABLE 241 GOOGLE: DEALS
- TABLE 242 BAIDU: BUSINESS OVERVIEW
- TABLE 243 BAIDU: PRODUCTS OFFERED
- TABLE 244 BAIDU: PRODUCT LAUNCHES
- TABLE 245 BAIDU: DEALS
- TABLE 246 AWS: BUSINESS OVERVIEW
- TABLE 247 AWS: PRODUCTS/SERVICES OFFERED
- TABLE 248 AWS: PRODUCT LAUNCHES
- TABLE 249 AWS: DEALS
- TABLE 250 AWS: OTHERS
- TABLE 251 ALTERYX: BUSINESS OVERVIEW
- TABLE 252 ALTERYX: PRODUCTS OFFERED
- TABLE 253 ALTERYX: PRODUCT LAUNCHES
- TABLE 254 ALTERYX: DEALS
- TABLE 255 HPE: BUSINESS OVERVIEW
- TABLE 256 HPE: PRODUCTS/SOLUTIONS OFFERED
- TABLE 257 HPE: PRODUCT LAUNCHES
- TABLE 258 HPE: DEALS
- TABLE 259 SALESFORCE: BUSINESS OVERVIEW
- TABLE 260 SALESFORCE: PRODUCTS/SOLUTIONS OFFERED
- TABLE 261 SALESFORCE: PRODUCT LAUNCHES
- TABLE 262 SALESFORCE: DEALS
- TABLE 263 ALTAIR: BUSINESS OVERVIEW
- TABLE 264 ALTAIR: PRODUCTS/SOLUTIONS OFFERED
- TABLE 265 ALTAIR: PRODUCT LAUNCHES
- TABLE 266 ALTAIR: DEALS
- TABLE 267 TERADATA: BUSINESS OVERVIEW
- TABLE 268 TERADATA: PRODUCTS/SOLUTIONS OFFERED
- TABLE 269 TERADATA: DEALS
- TABLE 270 H2O.AI: BUSINESS OVERVIEW
- TABLE 271 H2O.AI: PRODUCTS/SOLUTIONS OFFERED
- TABLE 272 H2O.AI: PRODUCT LAUNCHES
- TABLE 273 H2O.AI: DEALS
- TABLE 274 DATAROBOT: BUSINESS OVERVIEW
- TABLE 275 DATAROBOT: PRODUCTS/SERVICES OFFERED
- TABLE 276 DATAROBOT: DEALS
- TABLE 277 BIGML: BUSINESS OVERVIEW
- TABLE 278 BIGML: PRODUCTS/SOLUTIONS OFFERED
- TABLE 279 BIGML: PRODUCT LAUNCHES
- TABLE 280 BIGML: DEALS
- TABLE 281 DATABRICKS: BUSINESS OVERVIEW
- TABLE 282 DATABRICKS: PRODUCTS/SOLUTIONS OFFERED
- TABLE 283 DATABRICKS: PRODUCT LAUNCHES
- TABLE 284 DATABRICKS: DEALS
- TABLE 285 DATAIKU: BUSINESS OVERVIEW
- TABLE 286 DATAIKU: PRODUCTS/SOLUTIONS OFFERED
- TABLE 287 DATAIKU: PRODUCT LAUNCHES
- TABLE 288 DATAIKU: DEALS
- TABLE 289 MATHWORKS: BUSINESS OVERVIEW
- TABLE 290 MATHWORKS: PRODUCTS/SOLUTIONS OFFERED
- TABLE 291 MATHWORKS: PRODUCT LAUNCHES
- TABLE 292 MATHWORKS: DEALS
- TABLE 293 SPARKCOGNITION: BUSINESS OVERVIEW
- TABLE 294 SPARKCOGNITION: PRODUCTS/SOLUTIONS OFFERED
- TABLE 295 SPARKCOGNITION: PRODUCT LAUNCHES
- TABLE 296 SPARKCOGNITION: DEALS
- TABLE 297 QLIK: BUSINESS OVERVIEW
- TABLE 298 QLIK: PRODUCTS/SOLUTIONS OFFERED
- TABLE 299 QLIK: PRODUCT LAUNCHES
- TABLE 300 QLIK: DEALS
- TABLE 301 GLOBAL GENERATIVE AI MARKET SIZE AND GROWTH RATE, 2019–2022 (USD MILLION, Y-O-Y %)
- TABLE 302 GLOBAL GENERATIVE AI MARKET SIZE AND GROWTH RATE, 2023–2028 (USD MILLION, Y-O-Y %)
- TABLE 303 GENERATIVE AI MARKET, BY OFFERING, 2019–2022 (USD MILLION)
- TABLE 304 GENERATIVE AI MARKET, BY OFFERING, 2023–2028 (USD MILLION)
- TABLE 305 GENERATIVE AI MARKET, BY APPLICATION, 2019–2022 (USD MILLION)
- TABLE 306 GENERATIVE AI MARKET, BY APPLICATION, 2023–2028 (USD MILLION)
- TABLE 307 GENERATIVE AI MARKET, BY VERTICAL, 2019–2022 (USD MILLION)
- TABLE 308 GENERATIVE AI MARKET, BY VERTICAL, 2023–2028 (USD MILLION)
- TABLE 309 GENERATIVE AI MARKET, BY REGION, 2019–2022 (USD MILLION)
- TABLE 310 GENERATIVE AI MARKET, BY REGION, 2023–2028 (USD MILLION)
- TABLE 311 ARTIFICIAL INTELLIGENCE MARKET, BY OFFERING, 2016–2021 (USD BILLION)
- TABLE 312 ARTIFICIAL INTELLIGENCE MARKET, BY OFFERING, 2022–2027 (USD BILLION)
- TABLE 313 ARTIFICIAL INTELLIGENCE MARKET, BY TECHNOLOGY, 2016–2021 (USD BILLION)
- TABLE 314 ARTIFICIAL INTELLIGENCE MARKET, BY TECHNOLOGY, 2022–2027 (USD BILLION)
- TABLE 315 ARTIFICIAL INTELLIGENCE MARKET, BY DEPLOYMENT MODE, 2016–2021 (USD BILLION)
- TABLE 316 ARTIFICIAL INTELLIGENCE MARKET, BY DEPLOYMENT MODE, 2022–2027 (USD BILLION)
- TABLE 317 ARTIFICIAL INTELLIGENCE MARKET, BY ORGANIZATION SIZE, 2016–2021 (USD BILLION)
- TABLE 318 ARTIFICIAL INTELLIGENCE MARKET, BY ORGANIZATION SIZE, 2022–2027 (USD BILLION)
- TABLE 319 ARTIFICIAL INTELLIGENCE MARKET, BY BUSINESS FUNCTION, 2016–2021 (USD BILLION)
- TABLE 320 ARTIFICIAL INTELLIGENCE MARKET, BY BUSINESS FUNCTION, 2022–2027 (USD BILLION)
- TABLE 321 ARTIFICIAL INTELLIGENCE MARKET, BY VERTICAL, 2016–2021 (USD BILLION)
- TABLE 322 ARTIFICIAL INTELLIGENCE MARKET, BY VERTICAL, 2022–2027 (USD BILLION)
- TABLE 323 ARTIFICIAL INTELLIGENCE MARKET, BY REGION, 2016–2021 (USD BILLION)
- TABLE 324 ARTIFICIAL INTELLIGENCE MARKET, BY REGION, 2022–2027 (USD BILLION)
- FIGURE 1 AUTOMATED MACHINE LEARNING MARKET: RESEARCH DESIGN
- FIGURE 2 MARKET: TOP-DOWN AND BOTTOM-UP APPROACHES
- FIGURE 3 APPROACH 1 (SUPPLY SIDE): REVENUE FROM OFFERINGS OF MARKET PLAYERS
- FIGURE 4 APPROACH 2 – BOTTOM-UP (SUPPLY SIDE): COLLECTIVE REVENUE FROM OFFERINGS OF AUTOMATED MACHINE LEARNING MARKET PLAYERS
- FIGURE 5 APPROACH 3 – BOTTOM-UP (SUPPLY SIDE): REVENUE AND SUBSEQUENT MARKET ESTIMATION FROM MARKET OFFERINGS
- FIGURE 6 APPROACH 4 – BOTTOM-UP (DEMAND SIDE): SHARE OF AUTOMATED MACHINE LEARNING MARKET OFFERINGS THROUGH OVERALL AUTOMATED MACHINE LEARNING SPENDING
- FIGURE 7 SOLUTIONS SEGMENT TO LEAD MARKET IN 2023
- FIGURE 8 PLATFORMS SEGMENT TO ACCOUNT FOR LARGEST SHARE IN 2023
- FIGURE 9 OM-PREMISES SEGMENT TO ACCOUNT FOR LARGER SHARE DURING FORECAST PERIOD
- FIGURE 10 CONSULTING SERVICES SEGMENT TO ACCOUNT FOR LARGEST SHARE IN 2023
- FIGURE 11 DATA PROCESSING SEGMENT TO ACCOUNT FOR LARGEST SHARE IN 2023
- FIGURE 12 BFSI SEGMENT TO LEAD MARKET IN 2023
- FIGURE 13 NORTH AMERICA TO ACCOUNT FOR LARGEST SHARE IN 2023
- FIGURE 14 RISING DEMAND FOR PLATFORMS TO TRANSFER DATA FROM ON-PREMISES TO CLOUD TO DRIVE AUTOMATED MACHINE LEARNING MARKET
- FIGURE 15 RETAIL & ECOMMERCE SEGMENT TO ACCOUNT FOR LARGEST SHARE DURING FORECAST PERIOD
- FIGURE 16 NORTH AMERICA TO ACCOUNT FOR LARGEST SHARE BY 2028
- FIGURE 17 SOLUTIONS AND BFSI SEGMENTS TO ACCOUNT FOR SIGNIFICANT SHARE BY 2023
- FIGURE 18 AUTOMATED MACHINE LEARNING MARKET: DRIVERS, RESTRAINTS, OPPORTUNITIES, AND CHALLENGES
- FIGURE 19 ECOSYSTEM ANALYSIS
- FIGURE 20 AUTOMATED MACHINE LEARNING PIPELINE FRAMEWORK
- FIGURE 21 VALUE CHAIN ANALYSIS
- FIGURE 22 TOTAL NUMBER OF PATENTS GRANTED, 2021–2023
- FIGURE 23 TOP TEN COMPANIES WITH HIGHEST NUMBER OF PATENT APPLICATIONS, 2018–2021
- FIGURE 24 PORTER’S FIVE FORCES ANALYSIS
- FIGURE 25 INFLUENCE OF STAKEHOLDERS ON BUYING PROCESS FOR TOP THREE VERTICALS
- FIGURE 26 KEY BUYING CRITERIA FOR TOP THREE VERTICALS
- FIGURE 27 AUTOMATED MACHINE LEARNING MARKET: DISRUPTIONS IMPACTING BUYERS/CLIENTS
- FIGURE 28 SERVICES SEGMENT TO GROW AT HIGHER CAGR DURING FORECAST PERIOD
- FIGURE 29 PLATFORMS SEGMENT TO WITNESS HIGHER GROWTH DURING FORECAST PERIOD
- FIGURE 30 ON-PREMISES SEGMENT TO WITNESS HIGHER CAGR DURING FORECAST PERIOD
- FIGURE 31 TRAINING, SUPPORT, AND MAINTENANCE SEGMENT TO ACCOUNT FOR LARGEST SHARE DURING FORECAST PERIOD
- FIGURE 32 DATA PROCESSING SEGMENT TO LEAD MARKET DURING FORECAST PERIOD
- FIGURE 33 BFSI SEGMENT TO ACCOUNT FOR LARGER MARKET SIZE DURING FORECAST PERIOD
- FIGURE 34 ASIA PACIFIC TO GROW AT HIGHEST CAGR DURING FORECAST PERIOD
- FIGURE 35 INDIA TO GROW AT HIGHEST CAGR DURING FORECAST PERIOD
- FIGURE 36 NORTH AMERICA: MARKET SNAPSHOT
- FIGURE 37 ASIA PACIFIC: MARKET SNAPSHOT
- FIGURE 38 REVENUE ANALYSIS FOR KEY PLAYERS, 2018–2022
- FIGURE 39 MARKET SHARE ANALYSIS FOR KEY PLAYERS, 2022
- FIGURE 40 EVALUATION QUADRANT MATRIX FOR KEY PLAYERS, 2023
- FIGURE 41 EVALUATION QUADRANT MATRIX FOR SMES/STARTUPS, 2023
- FIGURE 42 COMPARATIVE ANALYSIS OF AUTOMATED MACHINE LEARNING PRODUCTS
- FIGURE 43 VALUATION AND FINANCIAL METRICS OF KEY AUTOMATED MACHINE LEARNING VENDORS
- FIGURE 44 YTD PRICE TOTAL RETURN AND STOCK BETA OF KEY AUTOMATED MACHINE LEARNING VENDORS
- FIGURE 45 IBM: COMPANY SNAPSHOT
- FIGURE 46 ORACLE: COMPANY SNAPSHOT
- FIGURE 47 MICROSOFT: COMPANY SNAPSHOT
- FIGURE 48 SERVICENOW: COMPANY SNAPSHOT
- FIGURE 49 GOOGLE: COMPANY SNAPSHOT
- FIGURE 50 BAIDU: COMPANY SNAPSHOT
- FIGURE 51 AWS: COMPANY SNAPSHOT
- FIGURE 52 ALTERYX: COMPANY SNAPSHOT
- FIGURE 53 HPE: COMPANY SNAPSHOT
- FIGURE 54 SALESFORCE: COMPANY SNAPSHOT
- FIGURE 55 ALTAIR: COMPANY SNAPSHOT
- FIGURE 56 TERADATA: COMPANY SNAPSHOT
The research methodology for the global AutoML market report involved the use of extensive secondary sources and directories, as well as various reputed open-source databases, to identify and collect information useful for this technical and market-oriented study. In-depth interviews were conducted with various primary respondents, including key opinion leaders, subject matter experts, high-level executives of multiple companies offering AutoML offerings, and industry consultants to obtain and verify critical qualitative and quantitative information, as well as assess the market prospects and industry trends.
Secondary Research
In the secondary research process, various secondary sources were referred to for identifying and collecting information for the study. The secondary sources included annual reports; press releases and investor presentations of companies; and white papers, certified publications, and articles from recognized associations and government publishing sources.
The secondary research was used to obtain key information about the industry’s value chain, the market’s monetary chain, the overall pool of key players, market classification and segmentation according to industry trends to the bottom-most level, regional markets, and key developments from both market and technology-oriented perspectives.
Primary Research
In the primary research process, various primary sources from both the supply and demand sides of the AutoML market ecosystem were interviewed to obtain qualitative and quantitative information for this study. The primary sources from the supply side included industry experts, such as chief executive officers (CEOs), vice presidents (VPs), marketing directors, technology and innovation directors, and related key executives from various vendors providing AutoML offerings; associated service providers; and system integrators operating in the targeted regions. All possible parameters that affect the market covered in this research study have been accounted for, viewed in extensive detail, verified through primary research, and analyzed to get the final quantitative and qualitative data.
After the complete market engineering (including calculations for market statistics, market breakup, market size estimations, market forecast, and data triangulation), extensive primary research was conducted to gather information and verify and validate the critical numbers arrived at. Primary research was also undertaken to identify and validate the segmentation types; industry trends; key players; the competitive landscape of the market; and key market dynamics, such as drivers, restraints, opportunities, challenges, industry trends, and key strategies.
In the complete market engineering process, both the top-down and bottom-up approaches were extensively used, along with several data triangulation methods, to perform the market estimation and market forecast for the overall market segments and subsegments listed in this report. Extensive qualitative and quantitative analysis was performed on the complete market engineering process to record the critical information/insights throughout the report.
The following is the breakup of primary profiles:
To know about the assumptions considered for the study, download the pdf brochure
Market Size Estimation
For making market estimates and forecasting the AutoML market and the other dependent submarkets, top-down and bottom-up approaches were used. The bottom-up procedure was used to arrive at the overall market size of the global AutoML market, using the revenue from the key companies and their offerings in the market. With data triangulation and validation through primary interviews, this study determined and confirmed the exact value of the overall parent market size. The overall market size was then used in the top-down procedure to estimate the size of other individual markets via percentage splits of the market segments.
The top-down approach prepared an exhaustive list of all the vendors offering AutoML. The revenue contribution of the market vendors was estimated through annual reports, press releases, funding and investor presentations, paid databases, and primary interviews. Each vendor’s offerings were evaluated based on the breadth of solution and service offerings, Applications, and verticals. The aggregate of all the companies’ revenues was extrapolated to reach the overall market size. Each subsegment was studied and analyzed for its global market size and regional penetration. The markets were triangulated through both primary and secondary research. The primary procedure included extensive interviews for key insights from industry leaders, such as CIOs, CEOs, VPs, directors, and marketing executives. The market numbers were further triangulated with the existing MarketsandMarkets repository for validation.
In the bottom-up approach, the adoption rate of AutoML solutions and services among different end-users in key countries with respect to their regions contributing the most to the market share was identified. For cross-validation, the adoption of automated machine learning solutions and services among industries, along with different use cases with respect to their regions, was identified and extrapolated. Weightage was given to use cases identified in different regions for the market size calculation.
All the possible parameters that affect the market covered in the research study have been accounted for, viewed in extensive detail, verified through primary research, and analyzed to get the final quantitative and qualitative data. The data is consolidated and added with detailed inputs and analysis from MarketsandMarkets.
- The pricing trend is assumed to vary over time.
- All the forecasts are made with the standard assumption that the accepted currency is USD.
- For the conversion of various currencies to USD, average historical exchange rates are used according to the year specified. For all the historical and current exchange rates required for calculations and currency conversions, the US Internal Revenue Service’s website is used.
- All the forecasts are made under the standard assumption that the globally accepted currency, USD, remains constant during the next five years.
- Vendor-side analysis: The market size estimates of associated solutions and services are factored in from the vendor side by assuming an average of licensing and subscription-based models of leading and innovative vendors.
- Demand/end-user analysis: End users operating in verticals across regions are analyzed in terms of market spending on Automted Machine Learning solutions based on some of the key use cases. These factors for the Automted Machine Learning tool industry per region are separately analyzed, and the average spending was extrapolated with an approximation based on assumed weightage. This factor is derived by averaging various market influencers, including recent developments, regulations, mergers and acquisitions, enterprise/SME adoption, startup ecosystem, IT spending, technology propensity and maturity, use cases, and the estimated number of organizations per region.
Data Triangulation
After arriving at the overall market size using the market size estimation processes as explained above, the market was split into several segments and subsegments. To complete the overall market engineering process and arrive at the exact statistics of each market segment and subsegment, data triangulation and market breakup procedures were employed, wherever applicable. The overall market size was then used in the top-down procedure to estimate the size of other individual markets via percentage splits of the market segmentation.
Market Definition
According to Microsoft, automated machine learning, also referred to as automated ML or AutoML, is the process of automating the time-consuming, iterative tasks of machine learning model development. It allows data scientists, analysts, and developers to build ML models with high scale, efficiency, and productivity all while sustaining model quality.
Key Stakeholders
- Research organizations
- Third-party service providers
- Technology providers
- Cloud services providers
- AI consulting companies
- Independent software vendors (ISVs)
- Service providers and distributors
- Application development vendors
- System integrators
- Consultants/consultancy/advisory firms
- Training and education service providers
- Support and maintenance service providers
- Managed service providers
Report Objectives
- To define, describe, and forecast the automated machine learning market based on offering, application, vertical, and region
- To provide detailed information about the major factors (drivers, restraints, opportunities, and challenges) influencing the market growth
- To analyze subsegments with respect to individual growth trends, prospects, and contributions to the total market
- To analyze opportunities in the market for stakeholders and provide the competitive landscape of the market
- To forecast the revenue of the market segments with respect to all the five major regions, namely, North America, Europe, Asia Pacific (APAC), the Middle East & Africa (MEA), and Latin America
- To profile the key players and comprehensively analyze the recent developments and their positioning related to the automated machine learning market
- To analyze competitive developments, such as mergers & acquisitions, product developments, and research & development (R&D) activities, in the market
- To analyze the impact of recession across all the regions across the automated machine learning market
Available Customizations
With the given market data, MarketsandMarkets offers customizations as per the company’s specific needs. The following customization options are available for the report:
Product Analysis
- Product matrix provides a detailed comparison of the product portfolio of each company
Geographic Analysis
- Further breakup of the North American market for Automted Machine Learning
- Further breakup of the European market for Automted Machine Learning
- Further breakup of the Asia Pacific market for Automted Machine Learning
- Further breakup of the Latin American market for Automted Machine Learning
- Further breakup of the Middle East & Africa market for Automted Machine Learning
Company Information
- Detailed analysis and profiling of additional market players (up to five)
Growth opportunities and latent adjacency in Automated Machine Learning (AutoML) Market