The Large Language Model (LLM) market research study involved extensive secondary sources, directories, journals, and paid databases. Primary sources were mainly industry experts from the core and related industries, preferred large language model providers, third-party service providers, consulting service providers, end users, and other commercial enterprises. In-depth interviews were conducted with various primary respondents, including key industry participants and subject matter experts, to obtain and verify critical qualitative and quantitative information, and assess the market’s prospects.
Secondary Research
In the secondary research process, various sources were referred to, for identifying and collecting information for this study. Secondary sources included annual reports, press releases, and investor presentations of companies; white papers, journals, and certified publications; and articles from recognized authors, directories, and databases. The data was also collected from other secondary sources, such as journals, government websites, blogs, and vendors websites. Additionally, large language model spending of various countries was extracted from the respective sources. Secondary research was mainly used to obtain key information related to the industry’s value chain and supply chain to identify key players based on solutions, services, market classification, and segmentation according to offerings of major players, industry trends related to software, hardware, services, technology, applications, warehouse sizes, verticals, and regions, and key developments from both market- and technology-oriented perspectives.
Primary Research
In the primary research process, various primary sources from both supply and demand sides were interviewed to obtain qualitative and quantitative information on the market. The primary sources from the supply side included various industry experts, including Chief Experience Officers (CXOs); Vice Presidents (VPs); directors from business development, marketing, and large language models expertise; related key executives from large language models solution vendors, SIs, professional service providers, and industry associations; and key opinion leaders.
Primary interviews were conducted to gather insights, such as market statistics, revenue data collected from solutions and services, market breakups, market size estimations, market forecasts, and data triangulation. Primary research also helped in understanding various trends related to technologies, applications, deployments, and regions. Stakeholders from the demand side, such as Chief Information Officers (CIOs), Chief Technology Officers (CTOs), Chief Strategy Officers (CSOs), and end users using large language models solutions, were interviewed to understand the buyer’s perspective on suppliers, products, service providers, and their current usage of large language models solutions and services, which would impact the overall large language model market.
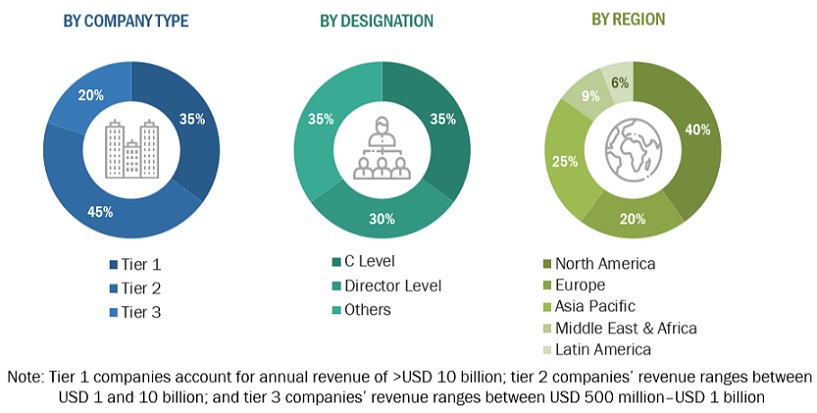
To know about the assumptions considered for the study, download the pdf brochure
Market Size Estimation
Multiple approaches were adopted for estimating and forecasting the large language model market. The first approach involves estimating the market size by summation of companies’ revenue generated through the sale of solutions and services.
Market Size Estimation Methodology-Top-down approach
In the top-down approach, an exhaustive list of all the vendors offering solutions and services in the large language model market was prepared. The revenue contribution of the market vendors was estimated through annual reports, press releases, funding, investor presentations, paid databases, and primary interviews. Each vendor's offerings were evaluated based on breadth of software and services according to data types, business functions, deployment models, and verticals. The aggregate of all the companies’ revenue was extrapolated to reach the overall market size. Each subsegment was studied and analyzed for its global market size and regional penetration. The markets were triangulated through both primary and secondary research. The primary procedure included extensive interviews for key insights from industry leaders, such as CIOs, CEOs, VPs, directors, and marketing executives. The market numbers were further triangulated with the existing MarketsandMarkets’ repository for validation.
Market Size Estimation Methodology-Bottom-up approach
In the bottom-up approach, the adoption rate of large language model solutions and services among different end users in key countries with respect to their regions contributing the most to the market share was identified. For cross-validation, the adoption of large language models solutions and services among industries, along with different use cases with respect to their regions, was identified and extrapolated. Weightage was given to use cases identified in different regions for the market size calculation.
Based on the market numbers, the regional split was determined by primary and secondary sources. The procedure included the analysis of the large language model market’s regional penetration. Based on secondary research, the regional spending on Information and Communications Technology (ICT), socio-economic analysis of each country, strategic vendor analysis of major large language models providers, and organic and inorganic business development activities of regional and global players were estimated. With the data triangulation procedure and data validation through primary interviews, the exact values of the overall large language model market size and segments’ size were determined and confirmed using the study.
Global Large Language Model Market Size: Bottom-Up and Top-Down Approach:
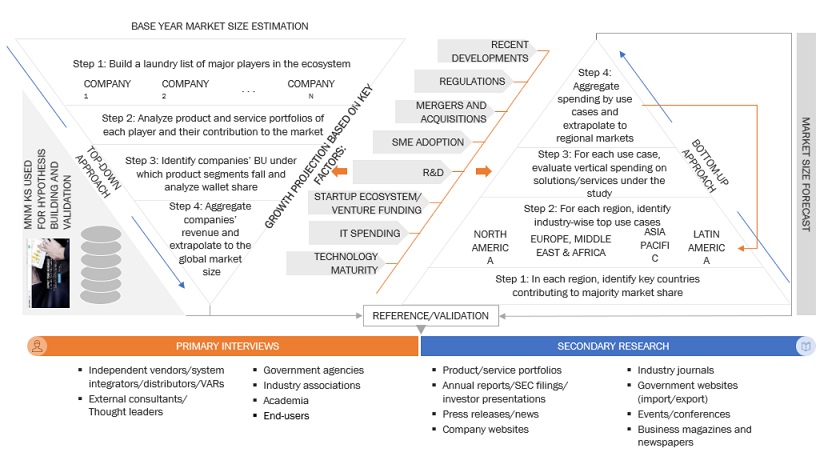
To know about the assumptions considered for the study, Request for Free Sample Report
Data Triangulation
After arriving at the overall market size using the market size estimation processes as explained above, the market was split into several segments and subsegments. To complete the overall market engineering process and arrive at the exact statistics of each market segment and subsegment, data triangulation and market breakup procedures were employed, wherever applicable. The overall market size was then used in the top-down procedure to estimate the size of other individual markets via percentage splits of the market segmentation.
Market Definition
A large language model (LLM) is a neural network-based artificial intelligence system trained on vast amounts of text data to understand and generate human-like language. It uses deep learning techniques to learn patterns and relationships, allowing coherent and contextually relevant text output. LLMs are called “large” as they consist of billions or trillions of parameters, enabling them to capture extensive knowledge and language patterns. This massive parameter count allows LLMs to perform a wide range of natural language processing tasks with human-like proficiency, such as text generation, summarization, translation, and question-answering.
Stakeholders
-
Generative AI software developers
-
Large language model software vendors
-
Business analysts
-
Cloud service providers
-
Consulting service providers
-
Enterprise end-users
-
Distributors and Value-added Resellers (VARs)
-
Government agencies
-
Independent Software Vendors (ISV)
-
Managed service providers
-
Market research and consulting firms
-
Support & maintenance service providers
-
System Integrators (SIs)/migration service providers
-
Language service providers
-
Technology providers
Report Objectives
-
To define, describe, and predict the large language model market by offering (software and services), architecture, modality, model size, application, end-user, and region
-
To provide detailed information related to major factors (drivers, restraints, opportunities, and industry-specific challenges) influencing the market growth
-
To analyze the micro markets with respect to individual growth trends, prospects, and their contribution to the total market
-
To analyze the opportunities in the market for stakeholders by identifying the high-growth segments of the market
-
To analyze opportunities in the market and provide details of the competitive landscape for stakeholders and market leaders
-
To forecast the market size of segments for five main regions: North America, Europe, Asia Pacific, Middle East Africa, and Latin America
-
To profile key players and comprehensively analyze their market rankings and core competencies.
-
To analyze competitive developments, such as partnerships, new product launches, and mergers and acquisitions, in the market
-
To analyze the impact of recession across all the regions across the large language model market
Available Customizations
With the given market data, MarketsandMarkets offers customizations as per your company’s specific needs. The following customization options are available for the report:
Product Analysis
-
Product quadrant, which gives a detailed comparison of the product portfolio of each company.
Geographic Analysis
-
Further breakup of the North American large language model market
-
Further breakup of the European market
-
Further breakup of the Asia Pacific market
-
Further breakup of the Middle Eastern & African market
-
Further breakup of the Latin America market
Company Information
-
Detailed analysis and profiling of additional market players (up to five)
Growth opportunities and latent adjacency in Large Language Model (LLM) Market