Generative AI in Pharmaceutical Market No Longer the Future
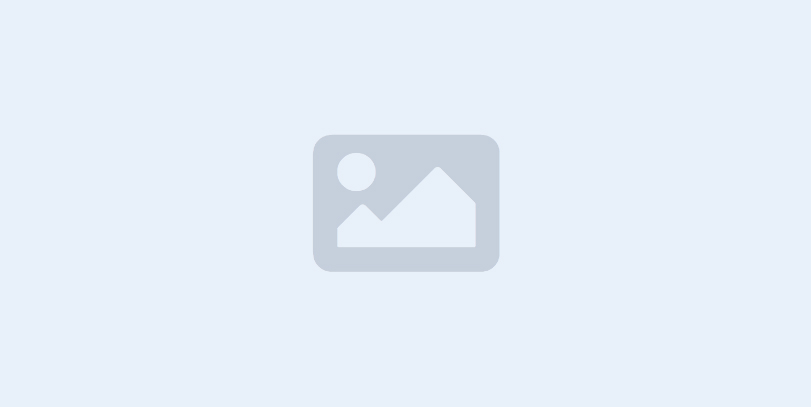
This News Covers
- How will generative AI disrupt the pharmaceutical market in near future?
- Alphabet, Nvidia, Astrazeneca and more: Generative AI and Pharmaceutical Ingredients?
- What are the top applications of generative AI in drug discovery and development?
- NVIDIA's role to get Generative AI advancements in Pharmaceuticals?
- How is generative AI transforming pharmaceutical research and development processes?
- What are the key cybersecurity implications and advancements driven by generative AI in the pharmaceutical sector?
- How does generative AI contribute to optimizing patient experiences and interface design in pharmaceutical products and services?
- What are the emerging trends and future prospects of generative AI in the pharmaceutical industry?
- How does generative AI impact data analytics and decision-making processes in pharmaceutical companies?
- What are the ethical considerations and potential risks associated with the use of generative AI in the pharmaceutical field?
- How is generative AI being utilized in the development of virtual reality (VR) and augmented reality (AR) technologies for pharmaceutical applications?
- What are the current and potential applications of generative AI in cloud computing and data management within the pharmaceutical industry?
Nvidia recently shared this development that Insilico Medicine has successfully used their AI platform to discover a drug candidate for idiopathic pulmonary fibrosis, one of the rare respiratory diseases and is now entering Phase 2 clinical trials.
By using generative AI, Insilico accomplished the preclinical drug discovery process in a fraction of the time and cost compared to traditional methods. The AI platform helped identify the target molecule, generate drug candidates, evaluate binding with the target, and predict clinical trial outcomes.
This achievement highlights the potential of AI-accelerated drug discovery. Insilico utilized NVIDIA Tensor Core GPUs and AI models trained on large datasets to power their generative AI drug design engine. The company's end-to-end approach combines deep learning for both biology and chemistry aspects of drug discovery. Insilico's success has led to a pipeline of promising drug candidates, demonstrating the effectiveness of generative AI systems in the field of drug discovery.
Generative AI is making significant contributions to cloud computing and data management in the pharmaceutical industry. It enables data analysis and insights, optimizing drug discovery and development, predictive analytics, personalized medicine, and treatment optimization. Combined with cloud computing, facilitates data integration and management, it is enabling real-time data processing and monitoring, optimized resource allocation. These applications enhance decision-making, improve operational efficiency, and are driving innovation in the pharmaceutical industry.
MarketsandMarkets welcomes this development and our editors share their views.
How will generative AI disrupt the pharmaceutical market in near future?
Generative AI is disrupting the pharmaceutical industry in several ways. Here are some key points:
- Content Generation and Personalization: Generative AI can produce new content, chat responses, designs, synthetic data, or deep fakes. This has the potential to revolutionize pharma marketing by changing the way companies market their products and allowing them to create content that is specifically tailored to the needs and preferences of individual patients based on patterns that previously could not be detected.
- Improved Compliance: Generative AI can improve compliance, which can be daunting when you consider the multitude of guidelines and regulations that exist in the pharma industry. The technology can serve as both a brainstorming bonanza at the outset of strategic development as well as the first pass to flag sensitive data or information to confirm that the content complies with defined legal requirements at the end.
- Automating Tasks: Generative AI can automate many of the laborious tasks that are currently done by humans, such as creating content, sending emails, and tracking leads. This can free up time for marketers and salespeople to focus on more strategic tasks, such as developing customer relationships and creating new marketing campaigns.
- Creating New Products and Services: Generative AI can help in tailoring products and services to the needs of individual users, greatly cutting down on the time to market without sacrificing crucial steps.
- Prediction: Generative AI can predict customer behavior, such as which products they are likely to buy and when they are likely to buy them. This can be used to create more targeted 1:1 marketing campaigns as well as to improve the customer experience in hours versus days.
However, the use of generative AI in marketing for pharma products raises a number of ethical concerns, including accuracy, bias, the perpetuation of inequality, and patient autonomy. It is important for companies and regulators to address these concerns and ensure that the use of generative AI in pharma marketing is ethical and responsible.
Alphabet, Nvidia, Astrazeneca and more: Generative AI and Pharmaceutical Ingredients?
Several major pharmaceutical companies are adopting generative AI technologies:
In addition to these, there are several AI in pharma suppliers such as Atomwise, BenevolentAI, Cyclica, Exscientia, Recursion, Iktos, Auransa, and InveniAI that are leading the way in the adoption of AI technologies in the pharmaceutical market.
- Alphabet: Alphabet recently launched Isomorphic Labs based on AI breakthroughs at its DeepMind AI operation.
- Nvidia: Nvidia has invested in the Clara suite of AI tools and applications.
- Baidu: Baidu's AI drug discovery unit has struck a major deal with Sanofi.
- AstraZeneca: AstraZeneca is spearheading the artificial intelligence hiring race, advertising for numerous new jobs related to artificial intelligence.
- Johnson & Johnson: Johnson & Johnson followed AstraZeneca as the second most proactive artificial intelligence employer, advertising for several new positions.
- Sanofi: Sanofi has partnered with Baidu's AI drug discovery unit.
What are the top applications of generative AI in drug discovery and development?
Generative AI has several key applications in drug discovery and development:
- Development of New Drugs: Generative AI can be used to create new chemical compounds that have the potential to be more effective and less harmful than existing drugs. It can also be used to design new drug delivery systems that can improve the efficacy and safety of drugs.
- Improving Efficiency of Drug Discovery: Generative AI can be used to screen large databases of chemical compounds to identify potential drug candidates. It can also be used to design experiments and analyze data, helping pharmaceutical companies discover new drugs more quickly and efficiently.
- Personalizing Treatments: Generative AI can be used to create models of the human genome and predict how patients will respond to different drugs. This information can be used to develop personalized treatment plans that are more effective and less harmful than traditional treatments.
- Pharma Marketing: Generative AI can revolutionize pharma marketing by changing the way companies market their products and allowing them to create content that is specifically tailored to the needs and preferences of individual patients based on patterns that previously could not be detected.
Here are some specific examples of how generative AI is being used in the pharmaceutical industry today:
- In 2020, a team of researchers from the University of California, San Francisco used generative AI to create a new drug that is effective against a type of cancer that is resistant to traditional treatments.
- In 2021, a team of researchers from the Massachusetts Institute of Technology used generative AI to design a new drug delivery system that can improve the efficacy and safety of cancer drugs.
- In 2022, a team of researchers from the University of Oxford used generative AI to create a personalized treatment plan for a patient with cystic fibrosis.
- In 2023, Insilico's utilization of generative AI was instrumental throughout the entire preclinical drug discovery process.
NVIDIA's role to get Generative AI advancements in Pharmaceuticals
Their AI platform enabled them to identify a molecule that could be targeted by a drug compound, generate novel drug candidates, assess the binding capabilities of these candidates with the target, and even predict the potential outcomes of clinical trials.
This achievement highlights the potential of AI-accelerated drug discovery. Insilico utilized NVIDIA Tensor Core GPUs and AI models trained on large datasets to power their generative AI drug design engine.
The traditional methods for accomplishing these tasks would have required a significant investment of over $400 million and up to six years of time. However, leveraging the power of generative AI, Insilico achieved these milestones at just one-tenth of the cost and one-third of the time, reaching the first phase of clinical trials in a remarkable two and a half years.
Moreover, Nvidia has introduced the BioNeMo Cloud Service, aimed at facilitating drug discovery by offering pre-trained AI models to drug researchers. This service aims to streamline the drug discovery cycle and enhance its efficacy. Companies like Amgen are currently utilizing the BioNeMo service.
How is generative AI transforming pharmaceutical research and development processes?
Generative AI is transforming pharmaceutical research and development processes in several ways:
- Accelerating Drug Discovery: Historically, creating a new medicine has been a time-consuming and resource-intensive endeavor. Researchers must navigate through an infinite number of compound possibilities before designing the best option. Once the compound is identified, scientists conduct testing procedures to ensure the treatment is effective, safe, and durable. With appropriate data and AI models, this process could be accelerated dramatically, saving valuable time and resources and eliminating the bias that comes with human trial and error.
- Data Collection and Analysis: For AI technology to be effective, it needs a robust data collection on the disease of interest, the proteins involved, how different molecules interact with those proteins, and much more. Companies like Sanofi are working to address this need for more data with their internal processes to achieve a more comprehensive integration of high-quality, diverse data, to enable the use of AI models in drug discovery. They are leveraging bioinformatics and omics data along with smart devices and wearables used in decentralized clinical trials to bolster their growing data libraries.
- Partnerships and Collaborations: Partnership and collaboration are key to achieving ambitious goals in AI and research innovation. Sanofi has announced several exciting collaborations with leading biotech companies emerging in the AI space. By leveraging their collaborations with partners like Owkin, Exscientia, Atomwise, and Insilico Medicine, they are already working to develop new ways to accelerate drug discovery and ultimately benefit patients more quickly.
- Developing New Therapies: AI is seen as the gateway to the next generation of therapies. Conditions in immunology and inflammation have been identified as areas of great opportunity where AI can offer game-changing aid in developing new treatments.
- Personalized Medicine: Generative AI can design personalized drugs optimized for specific patient populations. Generative AI can help improve treatment outcomes and reduce side effects by generating new molecules tailored to individual patient characteristics.
What are the key cybersecurity implications and advancements driven by generative AI in the pharmaceutical sector?
The key cybersecurity implications and advancements driven by generative AI in the pharmaceutical sector:
Implications
- Increased Vulnerability: The pharmaceutical industry is increasingly vulnerable to cyberattacks due to the sensitive and valuable data it holds, including information on patented drugs, advances, technologies, and sensitive patient information. Cyberattacks can lead to the weaponization of this data, eroding customer trust and brand goodwill.
- Rapid Digitalization: As the pharmaceutical industry adopts emerging technologies like IoT, big data, cloud computing, AI, machine learning, and blockchain, it faces new cybersecurity risks. While these technologies offer significant advantages, they also introduce potential vulnerabilities that need to be addressed.
- Regulatory Requirements: The pharmaceutical industry is subject to numerous regulatory requirements that mandate robust cybersecurity measures to protect personal data. Non-compliance can lead to severe penalties.
- Rising Cyber Threats: The industry faces a range of cybersecurity threats, including ransomware attacks, phishing scams, malware infections, social engineering tactics, insider threats, and supply chain attacks. These can result in data breaches, system downtime, critical data loss, and damage to the company's reputation.
Advancements:
- Generative AI Platforms: Platforms like ChatGPT rapidly analyze extensive data, identifying anomalies and patterns, enhancing cybersecurity defenses in pharmaceutical organizations.
- Increased Automation: AI fills workforce gaps in cybersecurity, requiring human oversight alongside its capabilities.
- Zero-Trust Approach: Adopting a zero-trust approach considers all users, devices, and applications potential threats, bolstering data, network, and access security.
- Third-Party Risk Management: Implementing robust programs for managing third-party risks adhering to cybersecurity standards reduces vulnerabilities associated with vendors accessing sensitive data and systems.
- Incident Response and Business Continuity Plans: Effective plans mitigate potential cyberattacks' impact, ensuring uninterrupted critical business functions.
The integration of robust cybersecurity infrastructure is crucial in the pharmaceutical industry, especially as it continues to evolve and adopt new technologies. This includes the use of generative AI, which can provide significant advancements in detecting and mitigating cybersecurity threats.
How does generative AI contribute to optimizing patient experiences and interface design in pharmaceutical products and services?
Generative AI contributes to optimizing patient experiences and interface design in pharmaceutical products and services in the following..
- Personalized Medicine: Generative AI can analyze large volumes of patient data, including medical records, genetic information, and lifestyle factors, to identify patterns and make personalized treatment recommendations. This enables pharmaceutical companies to develop tailored medicines and therapies that are more effective and specific to individual patients.
- Improved Patient Engagement: Generative AI can create interactive interfaces and chatbots that provide personalized support and guidance to patients. These interfaces can answer common questions, provide medication reminders, offer lifestyle recommendations, and enhance patient engagement throughout their healthcare journey.
- Enhanced User Experience: By leveraging generative AI algorithms, pharmaceutical companies can design user interfaces that are intuitive, user-friendly, and accessible. AI can analyze user behavior, preferences, and feedback to continuously improve the interface design, resulting in a seamless and positive user experience.
- Drug Discovery and Development: Generative AI algorithms can analyze vast amounts of scientific literature, research papers, and clinical trial data to identify potential drug targets and optimize the drug discovery process. This accelerates the development of new pharmaceutical products and improves the overall efficiency of the research and development pipeline.
- Adverse Event Detection: Generative AI can analyze patient data, electronic health records, and social media posts to detect and monitor adverse drug reactions. By identifying potential side effects and adverse events early on, pharmaceutical companies can take proactive measures to ensure patient safety and improve the overall quality of their products.
- Precision Marketing: Generative AI can analyze patient demographics, preferences, and behavior to develop targeted marketing campaigns. By understanding patient needs and tailoring marketing messages accordingly, pharmaceutical companies can effectively communicate the benefits of their products and services to the intended audience.
- Real-Time Monitoring and Feedback: Generative AI algorithms can collect and analyze real-time patient data, such as vital signs, symptoms, and treatment responses. This enables healthcare providers to monitor patients remotely, provide timely interventions, and gather valuable feedback to improve treatment outcomes and patient experiences.
What are the emerging trends and future prospects of generative AI in the pharmaceutical industry?
- Drug Discovery and Development: Revolutionizing the process by predicting drug-target interactions, identifying therapeutic candidates, and optimizing drug design through data analysis.
- Precision Medicine: Tailoring treatment plans and optimizing drug response based on patient data and genetic information, enabling personalized medicine.
- Optimizing Clinical Trials: Streamlining trial processes by identifying suitable patient populations, predicting outcomes, and optimizing trial design for cost reduction and improved efficiency.
- Virtual Drug Screening: Simulating and predicting drug effects on target proteins to expedite identification of potential candidates and reduce experimental screening time and cost.
- Real-Time Monitoring and Patient Care: Enabling personalized and proactive healthcare interventions through real-time monitoring of vital signs, symptoms, and treatment responses.
- Supply Chain Optimization: Optimizing inventory, logistics, and demand prediction in the pharmaceutical supply chain to enhance efficiency and reduce costs.
- Enhanced Decision-Making: Providing insights and predictive analytics for informed decision-making in treatment plans and research directions.
- Ethical Considerations and Regulatory Framework: Addressing challenges such as data quality, transparency, interpretability, and regulatory compliance for responsible and accountable use of generative AI in healthcare.
How does generative AI impact data analytics and decision-making processes in pharmaceutical companies?
Generative AI empowers pharmaceutical companies to leverage their data effectively, gain valuable insights, and make informed decisions throughout the drug discovery and development process. It enhances the speed, accuracy, and efficiency of data analytics, leading to improved decision-making and ultimately benefiting patients by delivering better treatments and healthcare outcomes.
- Data Analysis: Analyzing large volumes of diverse data to extract insights, identify patterns, and discover new relationships.
- Drug Discovery: Data to identify drug targets, predict interactions, and optimize molecule design for faster and more efficient discovery.
- Precision Medicine: Patient data to tailor treatment plans, optimize drug response, and personalize medicine.
- Rare Disease Identification: Symptoms, genetics, and medical records to detect and diagnose rare diseases earlier.
- Clinical Trial Optimization: Optimizing trial design, patient selection, and protocols for efficient and cost-effective trials.
- Predictive Analytics: Analyzing historical data to predict trends, outcomes, and inform decision-making.
- Risk Assessment and Safety Monitoring: Assessing drug safety through adverse event analysis and real-world evidence.
- Resource Allocation: Optimizing resource allocation based on demand, logistics, and market dynamics for cost savings and operational efficiency.
What are the ethical considerations and potential risks associated with the use of generative AI in the pharmaceutical field?
It is important for pharmaceutical companies and stakeholders to address these ethical considerations and potential risks associated with the use of generative AI. By implementing responsible AI practices, promoting transparency, and prioritizing patient well-being, the benefits of generative AI can be maximized while mitigating potential harms.
Ethical Considerations:
- Data Privacy and Security: The use of generative AI involves handling large volumes of sensitive patient data, including medical records and genetic information. Ensuring data privacy and implementing robust security measures is essential to protect patient confidentiality and prevent unauthorized access or data breaches.
- Algorithm Bias and Fairness: Generative AI algorithms are trained on existing data, which may contain biases and reflect existing healthcare disparities. This can result in biased predictions and decisions, leading to unequal access to healthcare resources and exacerbating existing inequities. Efforts should be made to address algorithmic bias and ensure fairness in the use of generative AI.
- Transparency and Explainability: Generative AI algorithms can be complex and difficult to interpret. Ensuring transparency and explainability of AI algorithms is crucial to understand how decisions are made and to gain trust from patients, healthcare professionals, and regulatory authorities.
- Informed Consent and Data Governance: The use of patient data in generative AI requires informed consent and adherence to ethical guidelines for data governance. Patients should be informed about how their data will be used, and proper consent processes should be in place to ensure the ethical and responsible use of data.
Potential Risks:
- Dissemination of False Information: The use of generative AI in pharmaceutical marketing may increase the risk of disseminating false or misleading information. AI-generated content needs to be carefully monitored and verified to ensure accuracy and prevent the spread of misinformation that could negatively impact patient well-being.
- Lack of Human Oversight: Overreliance on generative AI without proper human oversight can lead to errors or incorrect conclusions. Human expertise and judgment are essential to validate AI-generated insights and make informed decisions.
- Unintended Consequences: The use of generative AI may have unintended consequences or unforeseen impacts on patient outcomes, treatment decisions, and healthcare systems. Continuous monitoring and evaluation are necessary to identify and address any negative consequences that may arise.
- Regulatory and Legal Compliance: The use of generative AI in the pharmaceutical field must comply with existing regulatory frameworks and legal requirements. This includes adherence to data protection laws, patient privacy regulations, and ethical guidelines for research and development.
- Lack of Standardization and Quality Control: The quality and reliability of generative AI algorithms can vary. Lack of standardization and quality control measures may result in inconsistent performance and inaccurate predictions. Ensuring robust validation, verification, and quality control processes are in place is essential.
How is generative AI being utilized in the development of virtual reality (VR) and augmented reality (AR) technologies for pharmaceutical applications?
Generative AI is being utilized in the development of virtual reality (VR) and augmented reality (AR) technologies for pharmaceutical applications in the following ways..
- Simulation and Training: Generative AI algorithms can create realistic virtual environments and scenarios for training healthcare professionals, including pharmacists and clinicians. VR and AR technologies combined with generative AI can simulate medical procedures, drug interactions, and patient consultations, providing a safe and immersive training experience.
- Product Visualization: Generative AI algorithms can generate virtual representations of pharmaceutical products, allowing users to visualize and interact with them in virtual or augmented reality. This enables pharmaceutical companies to showcase their products, such as drugs, medical devices, or packaging, in a more engaging and informative way.
- Patient Education and Engagement: VR and AR, powered by generative AI, can provide immersive educational experiences for patients. Virtual environments can be created to explain complex medical concepts, demonstrate the mode of action of drugs, or showcase treatment procedures. This enhances patient understanding, engagement, and adherence to prescribed medications.
- Clinical Trials and Data Analysis: Generative AI algorithms can analyze data from clinical trials and generate visualizations in VR or AR environments. This enables researchers and healthcare professionals to explore and interpret complex data sets more effectively, identify patterns, and gain insights that can inform decision-making in clinical research and drug development.
- Remote Collaboration and Telemedicine: VR and AR technologies combined with generative AI can facilitate remote collaboration and telemedicine. Healthcare professionals can use virtual environments to interact with patients in real-time, provide remote consultations, and visualize patient data, enhancing the delivery of healthcare services.
- Medical Marketing and Sales: VR and AR, powered by generative AI, can enhance medical marketing and sales efforts. Pharmaceutical companies can create interactive product demonstrations, virtual tours of manufacturing facilities, or immersive experiences showcasing the benefits of their products. This helps engage customers and provide them with a qualitative experiential understanding of the pharmaceutical products.
By leveraging generative AI in conjunction with VR and AR technologies, the pharmaceutical industry can unlock new possibilities in training, education, data analysis, remote collaboration, and marketing. These technologies enhance user experiences, improve healthcare outcomes, and drive innovation in pharmaceutical applications.
What are the current and potential applications of generative AI in cloud computing and data management within the pharmaceutical industry?
The combination of generative AI and cloud computing in data management and analysis offers significant potential for transforming various aspects of the pharmaceutical industry, from drug discovery to personalized medicine and resource optimization.
- Data Analysis and Insights: Generative AI algorithms can analyze large volumes of pharmaceutical data, including electronic health records, clinical trial data, and genomic data, to extract valuable insights. This can help pharmaceutical companies identify patterns, predict outcomes, and make data-driven decisions in drug discovery, development, and patient care.
- Drug Discovery and Development: Generative AI can optimize the drug discovery process by analyzing vast amounts of scientific literature, research papers, and clinical trial data. It can assist in identifying potential drug targets, optimizing molecular structures, predicting drug efficacy, and accelerating the discovery and development of new pharmaceutical products.
- Predictive Analytics and Risk Assessment: Generative AI can leverage cloud computing resources to perform predictive analytics and risk assessment in the pharmaceutical industry. By analyzing historical data and utilizing machine learning algorithms, it can predict patient outcomes, identify potential adverse events, and assess the safety and efficacy of drugs.
- Personalized Medicine and Treatment Optimization: Generative AI algorithms can utilize cloud computing capabilities to analyze patient data, including genetic information and medical records, to enable personalized medicine. This includes identifying biomarkers, predicting treatment response, and optimizing treatment plans based on individual patient characteristics.
- Data Integration and Management: Cloud-based generative AI platforms can assist in integrating and managing diverse data sources within the pharmaceutical industry. This includes consolidating data from various systems, ensuring data quality and consistency, and providing secure access and sharing of data among different stakeholders.
- Real-Time Data Processing and Monitoring: Cloud-based generative AI can enable real-time data processing and monitoring in pharmaceutical applications. This includes monitoring patient health data, analyzing sensor data from medical devices, and providing timely insights and alerts for healthcare professionals.
- Optimized Resource Allocation and Efficiency: Generative AI algorithms, combined with cloud computing capabilities, can optimize resource allocation and improve operational efficiency in the pharmaceutical industry. This includes managing inventory, optimizing supply chain logistics, and reducing costs through predictive demand forecasting and production planning.
- Collaboration and Knowledge Sharing: Cloud-based generative AI platforms facilitate collaboration and knowledge sharing among researchers, scientists, and healthcare professionals. They enable seamless access to shared datasets, collaborative analysis, and the exchange of insights and findings, promoting innovation and advancement in the pharmaceutical field.
References:
GET AHEAD
Top Research Reports to Fuel Your Industry Knowledge- Artificial Intelligence (AI) Market by Offering (Hardware, Software), Technology (ML (Deep Learning (LLM, Transformers (GPT 1, 2, 3, 4)), NLP, Computer Vision), Business Function, Vertical, and Region - Global Forecast to 2030
- Pharmaceutical Manufacturing Equipment Market by Equipment Type (Packaging, Mixing & Blending, Filling, Milling, Spray Drying, Inspection, Extrusion, Tablet Compression Presses), End-Product Type (Solid, Liquid) and Region - Global Forecast to 2028
Editor's Pick
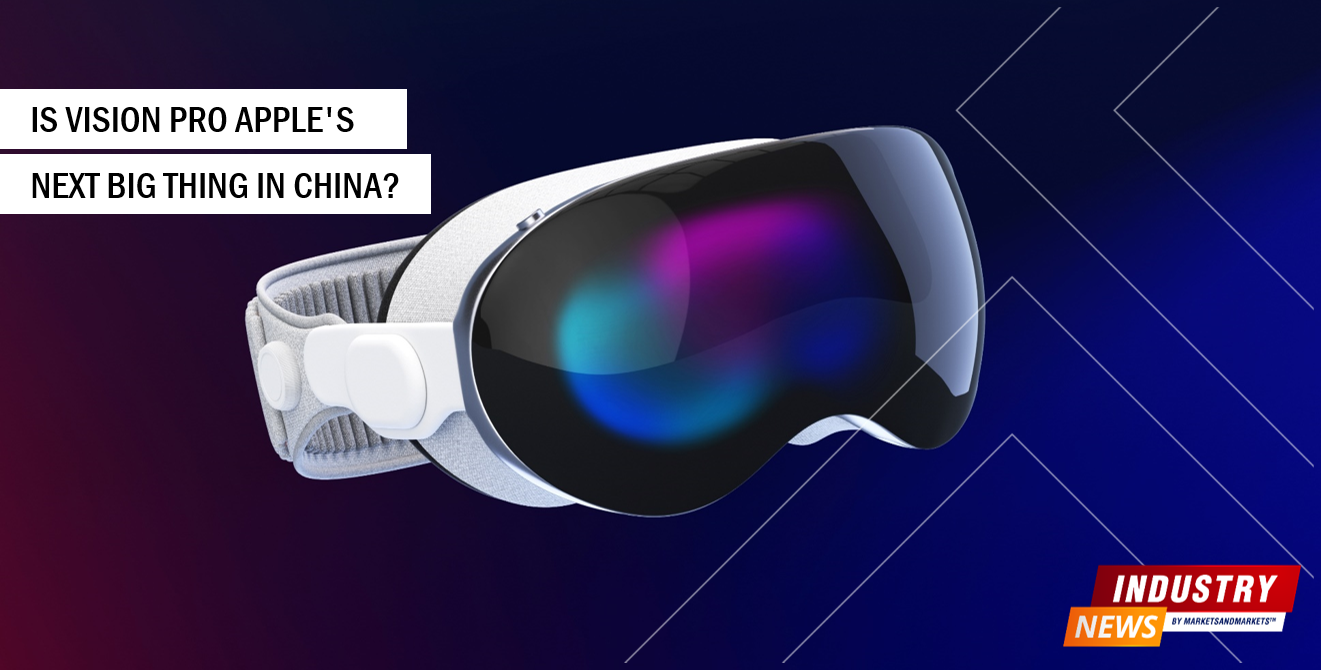
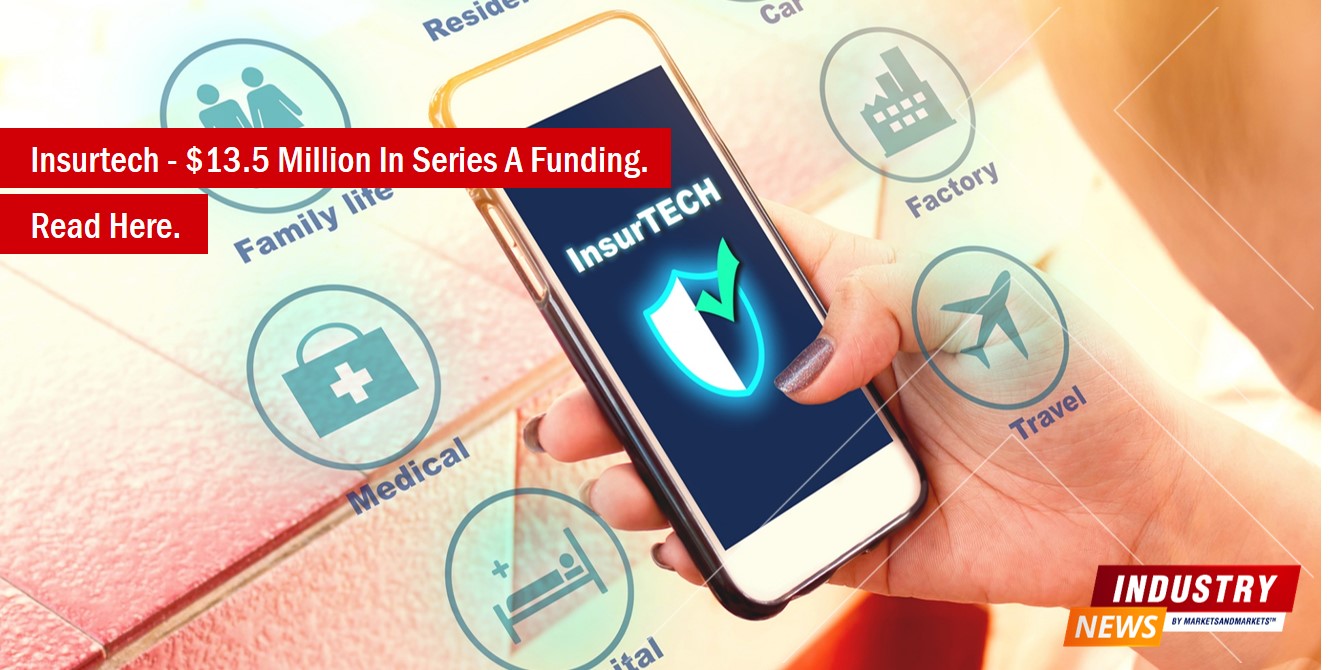
Information and Communication Technology
Insurtech Funding News - Coverdash raises USD 13.5 Million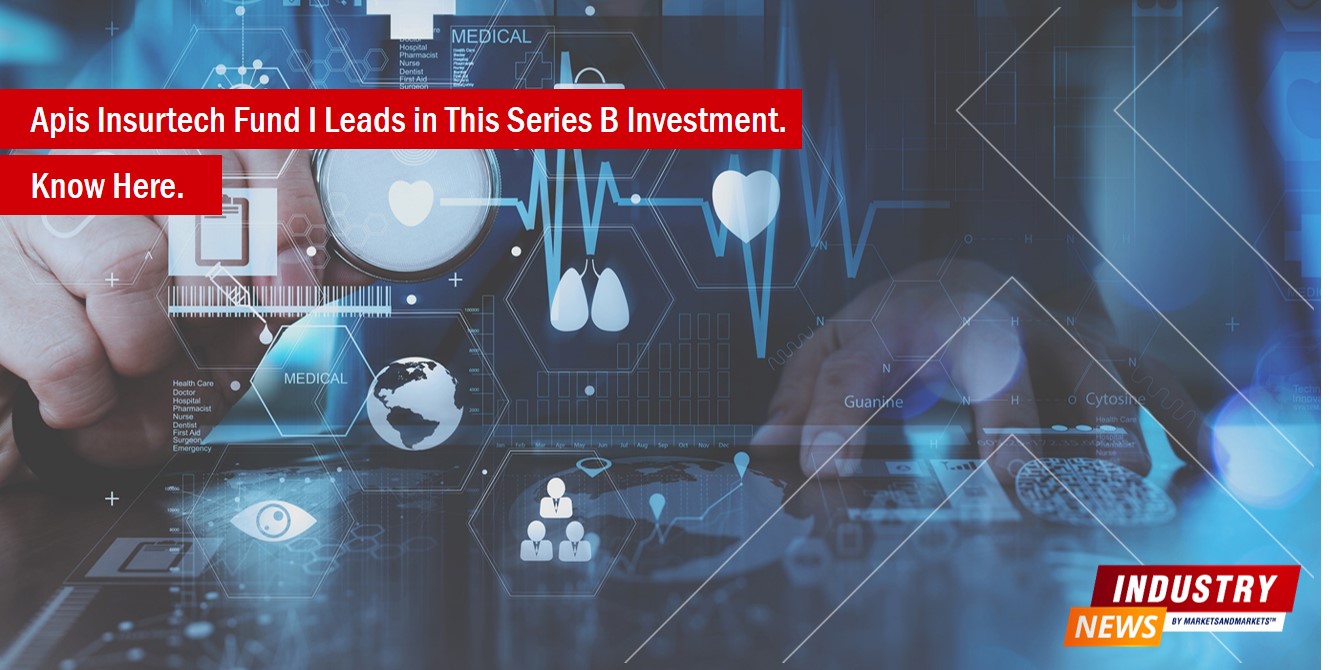
PODCASTS
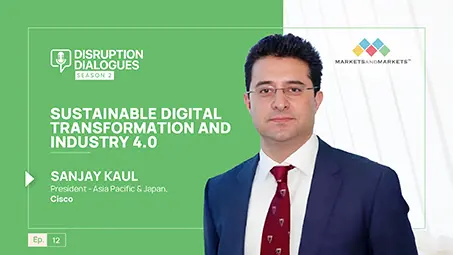
Sustainable Digital Transformation & Industry 4.0
Sanjay Kaul, President-Asia Pacific & Japan, Cisco, and host Aashish Mehra, Chief Research Officer, MarketsandMarkets, in conversation on unraveling 'Sustainable Digital Transformation and Industry 4.0'
11 July 2023|S2E12|Listen Now
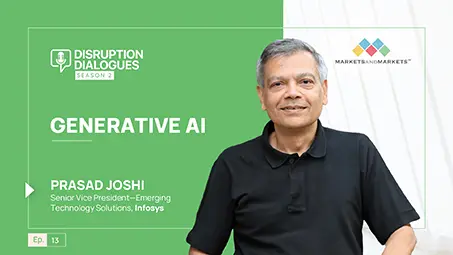
Generative AI
Prasad Joshi, Senior Vice President-Emerging Technology Solutions, Infosys, and host, Vinod Chikkareddy, CCO, MarketsandMarkets, in exploring the recent advances in AI and the generative AI space.
7 Nov 2023|S2E13|Listen Now
Download Whitepaper
